Using Fecal DNA and Spatial Capture–Recapture to
Characterize a Recent Coyote Colonization
Christine A. Bozarth, Beth Gardner, Larry L. Rockwood, and Jesús E. Maldonado
Northeastern Naturalist, Volume 22, Issue 1 (2015): 144–162
Full-text pdf (Accessible only to subscribers. To subscribe click here.)

Access Journal Content
Open access browsing of table of contents and abstract pages. Full text pdfs available for download for subscribers.
Current Issue: Vol. 30 (3)

Check out NENA's latest Monograph:
Monograph 22
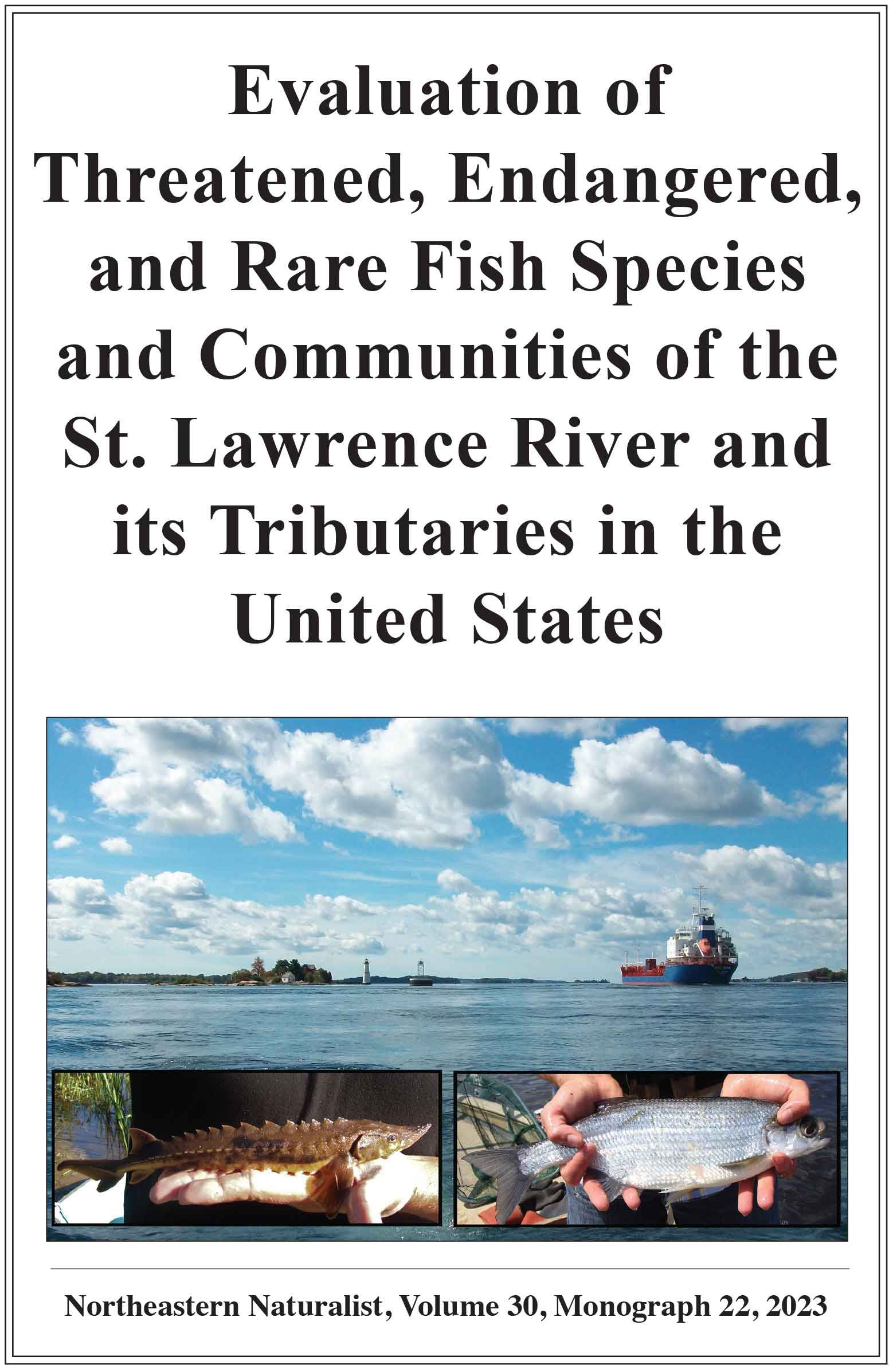
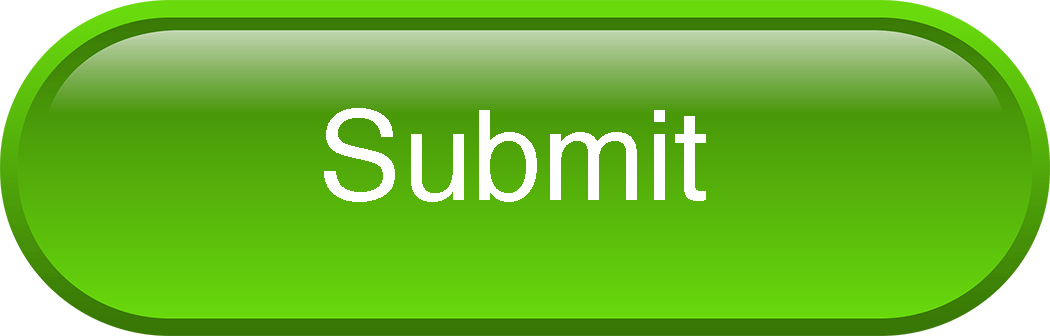
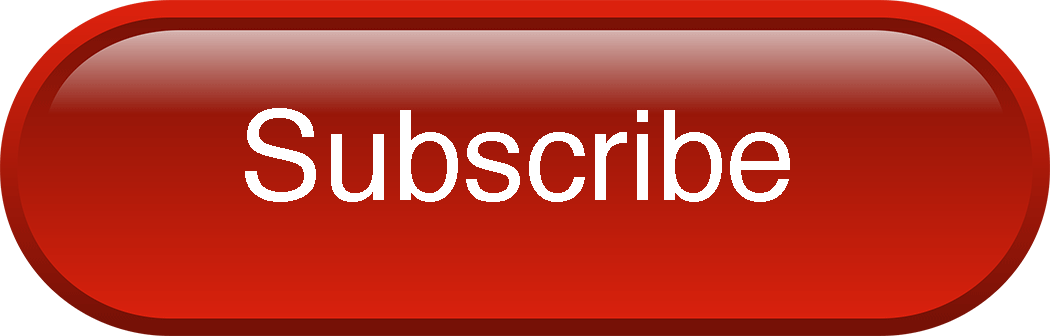
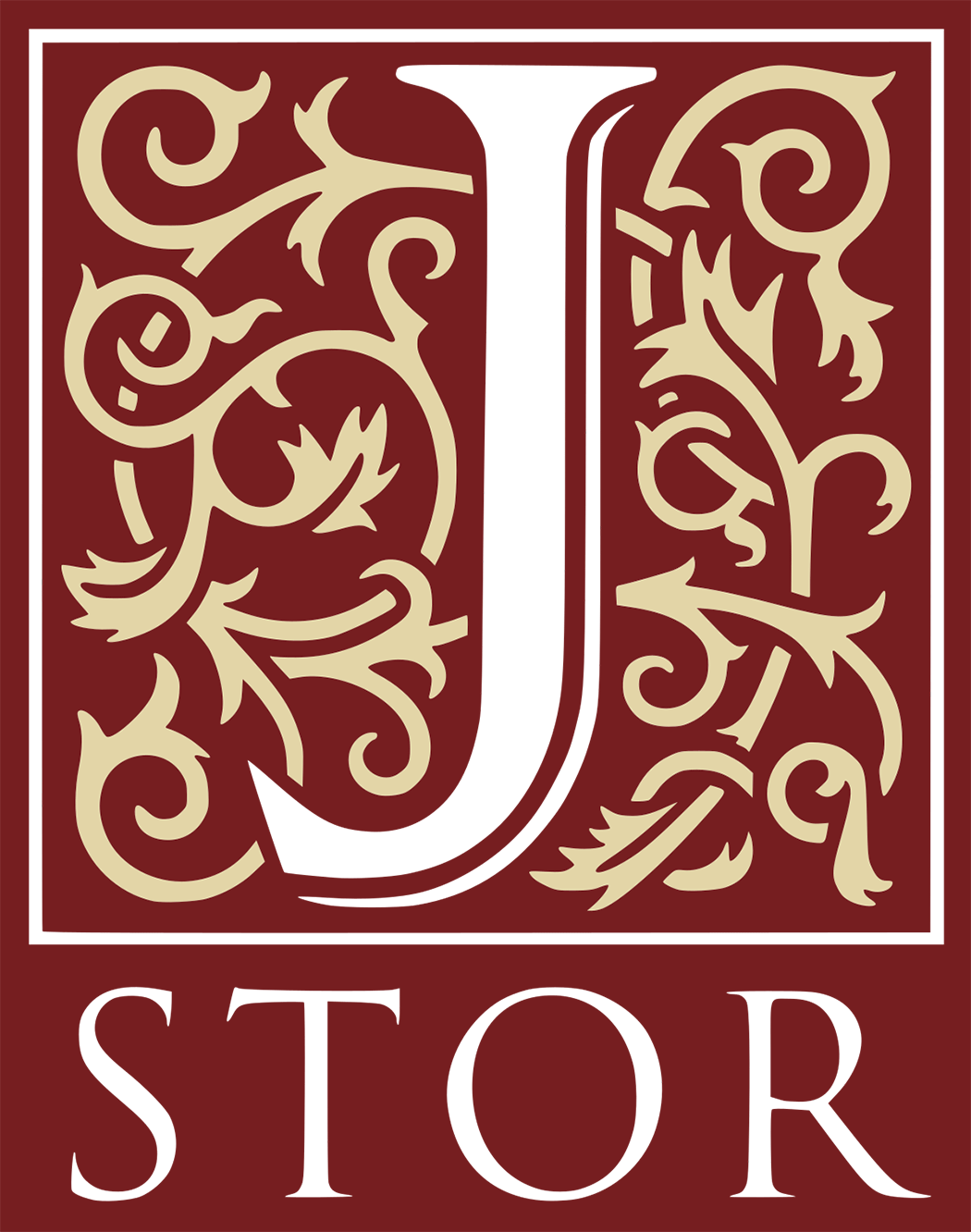
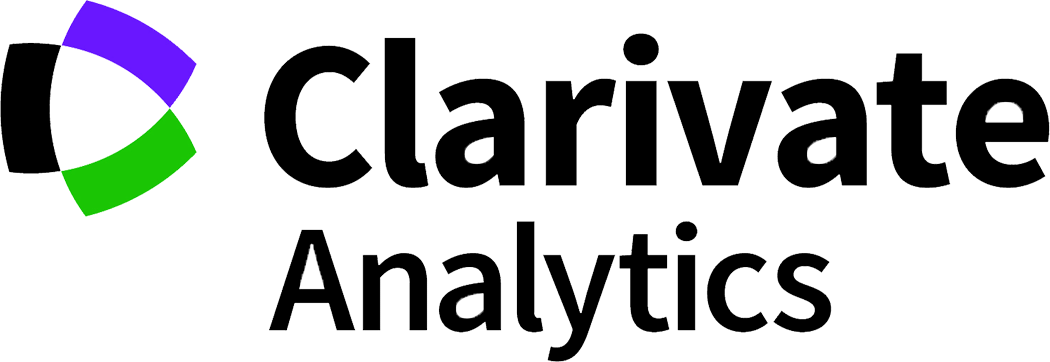
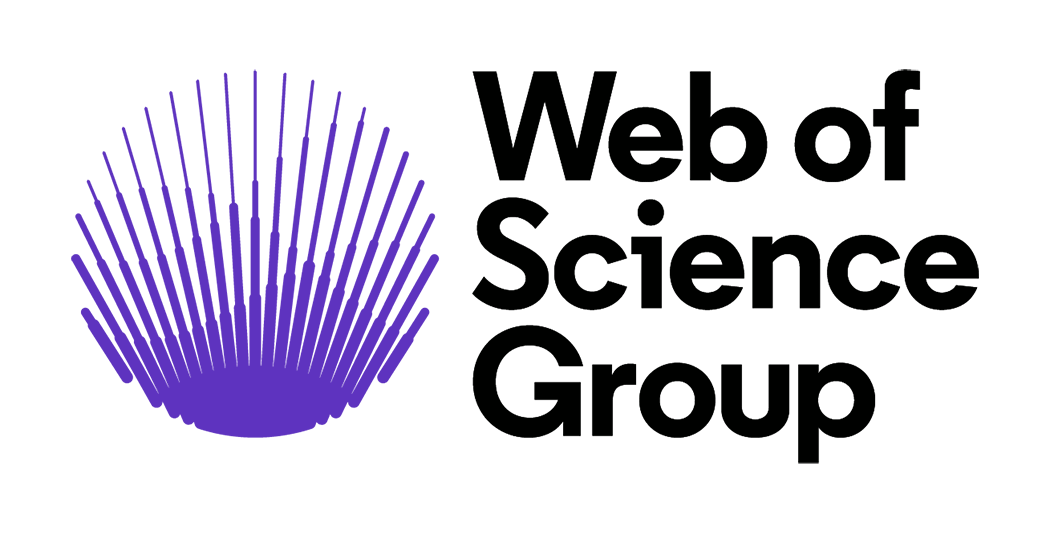
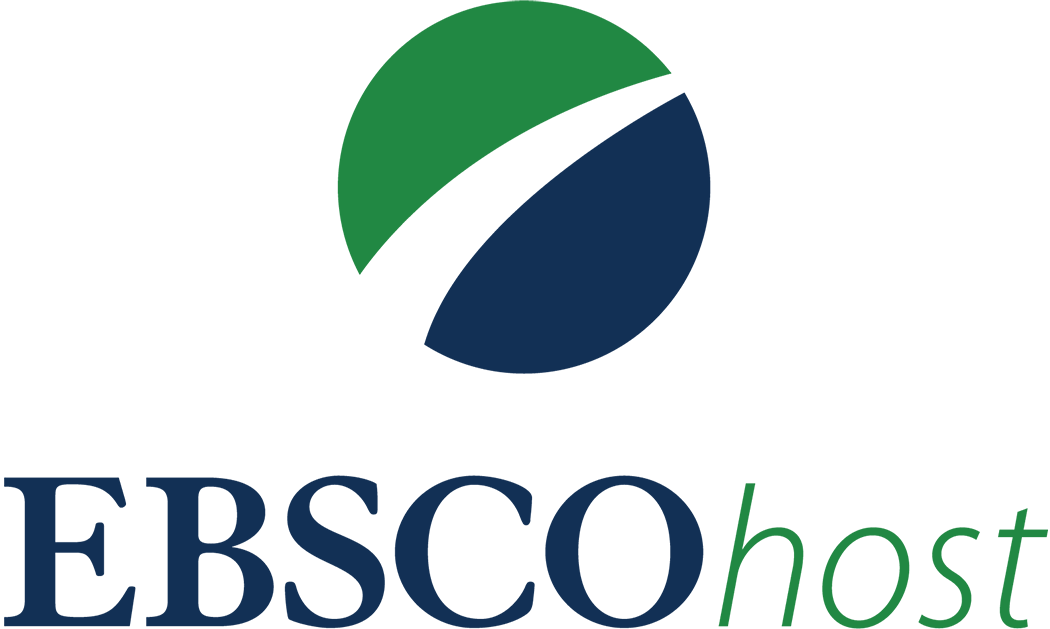
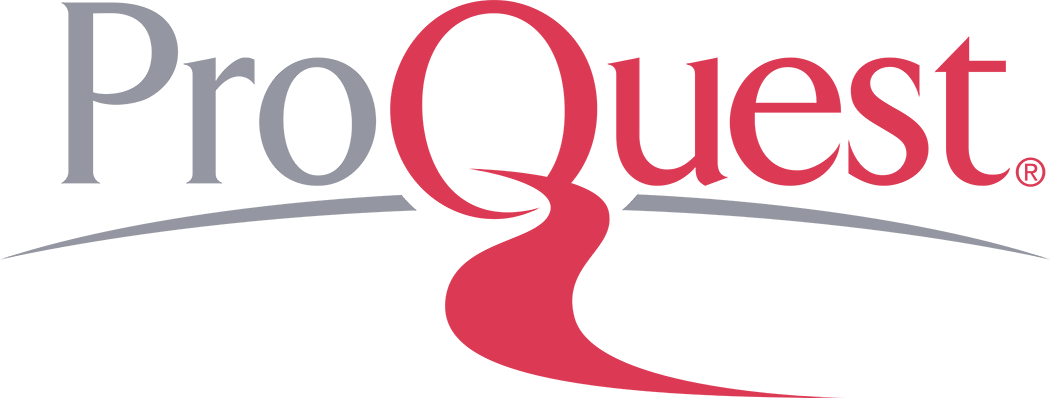
Northeastern Naturalist
144
C.A. Bozarth, B. Gardner, L.L. Rockwood, and J.E. Maldonado
22001155 NORTHEASTERN NATURALIST 2V2(o1l). :2124,4 N–1o6. 21
Using Fecal DNA and Spatial Capture–Recapture to
Characterize a Recent Coyote Colonization
Christine A. Bozarth1, 2,*, Beth Gardner3, Larry L. Rockwood1, 2, and
Jesús E. Maldonado1, 2, 4
Abstract - The arrival of a novel predator in an ecosystem necessitates many wildlifemanagement
decisions that should be based on sound demographic data. Canis latrans
(Coyote) has experienced a dramatic range expansion across North America since the early
19th century, completing its colonization of the continental US in the mid-Atlantic region
over the past 20 years. Their arrival in the suburbs of Washington, DC, has generated much
public attention, and demonstrated a need for demographic information about this species.
To address the challenges of surveying an elusive animal, we used fecal DNA to describe the
population genetics and demographics of a newly colonized Coyote population at Marine
Corps Base Quantico (MCBQ) in northern Virginia. We collected 331 scats over a period
of 2 years at MCBQ, resulting in identification of 23 unique individual Coyotes and 41
total Coyote captures that were analyzed using spatial capture–recapture models. We found
evidence of colonization by multiple genetic lineages and a low population density of 0.047
individuals/km2. Importantly, this study incorporates a new class of models on individual
animals identified by genotype data derived from fecal DNA and demonstrates the utility of
these models in surveying elusive animals.
Introduction
When a new species colonizes an area, estimating demographic parameters is
often the first step in designing an effective wildlife-management strategy. Canis
latrans Say (Coyote) has experienced a dramatic range expansion in eastern North
America over the past 100 years (Parker 1995). East of the Mississippi River, they
have colonized eastward along 2 general routes: a northern route moving across
the Great Lakes region and into the northeastern US and a southern route moving
through the southeastern US. Based on reports of Coyote presence from both primary
literature and state and federal wildlife agencies (Parker 1995, Mastro 2011),
these 2 fronts appear to converge along the Appalachian Mountains and in the
mid-Atlantic region (Delaware, the District of Columbia, Maryland, and Virginia).
The mid-Atlantic region is the last area to have been colonized by Coyotes in the
continental US; the animals were first detected in the area as late as 1993 (Parker
1Center for Conservation and Evolutionary Genetics, Smithsonian Conservation Biology
Institute, Washington, DC 20008. 2Department of Environmental Science and Policy,
George Mason University, Fairfax, VA 22030. 3Fisheries, Wildlife, and Conservation Biology
Program, Department of Forestry and Environmental Resources, North Carolina State
University, Raleigh, NC 27695. 4Department of Vertebrate Zoology, National Museum of
Natural History, Smithsonian Institution, Washington, DC 20013. *Corresponding author -
cbozarth@nvcc.edu.
Manuscript Editor: Adrienne Kovach
Northeastern Naturalist Vol. 22, No. 1
C.A. Bozarth, B. Gardner, L.L. Rockwood, and J.E. Maldonado
2015
145
1995). Northern Virginia is at the heart of this region; it is loosely defined and
consists of several counties and independent cities radiating to the south and west
of Washington, DC. This area has a dense human population and has experienced
massive human development in the past decade (GMUCRA 2010). Public opinion
regarding Coyote presence in Northern Virginia has been charged and polarized,
with residents noting the need for more information on the new colonists (Battiata
2006). There have been many genetic, ecological, behavioral, and management
studies on Coyotes throughout much of North America (e.g., Gehrt and Prange
2007, Hailer and Leonard 2008, Kamler and Gipson 2000, Kays et al. 2008, Prugh
et al. 2005, Riley et al. 2006, Sacks et al. 2008, Way et al. 2010, Wheeldon et al.
2010), but very limited research has been conducted in the mid-Atlantic States (but
see Bozarth et al. 2011 and Mastro 2011), despite its location as a convergence zone
between two waves of colonization.
Non-invasive molecular methods provide a fast and reliable way to characterize
a population of an elusive species. Fecal DNA analysis has been used to examine
population demographics of several carnivore species, including Lynx rufus (Schreber)
(Bobcat; Ruell et al. 2009), Ursus arctos L. (Brown Bear; Bellemain et al.
2005), Coyote (Kohn et al. 1999, Prugh et al. 2005), Canis lupus L. (Gray Wolf;
Lucchini et al. 2002, Valière et al. 2003), Vulpes macrotis Merriam (Kit Fox; Smith
et al. 2006), Puma concolor L. (Puma; Miotto et al. 2007), and Gulo gulo (Wolverine;
Flagstad et al. 2004). Demographic parameters such as relatedness, population
size, and genetic structure can be obtained without the need to capture animals, thus
eliminating potential stress for the animals and modification of their natural behavior
(Archie et al. 2007, 2008; Spiering et al. 2010). Coyotes are ideal candidates for
non-invasive study because they can be difficult to trap but tend to defecate on roads
and trails which makes their feces (scat) easy to detect (Macdonald 1980).
The detection of large carnivores for estimating population demographic parameters
can be hampered by 2 major issues: the violation of assumption of population
closure due to their large home ranges, and sparse data sets due to their elusiveness.
Estimates of home range for Coyotes with established territories vary from an
average of 4.1 km2 in northeastern Kansas (Kamler and Gipson 2000) to 46.4 km2
in eastern Maine (Harrison et al. 1989). Home-range sizes for transient Coyotes
range from an average of only 12.4 km2 in southern Texas (Windberg and Knowlton
1988) to 219.7 km2 in southern British Columbia (Atkinson and Shackleton 1991).
In populations with large home-ranges, individual animals may move into and out
of the study site, thus influencing estimates of detection probability and abundance
(Efford 2004, Gardner et al. 2009, Royle and Young 2008). In addition to potential
violations of population-closure assumptions, large carnivores can be difficult to
detect, resulting in small and incomplete data sets that may bias demographic estimates
(Smallwood and Schonewald 1998). Population models that are not spatially
explicit rely on a variety of ad hoc methods to buffer the study area to account for
individual movements outside of the area instead of incorporating spatial data in
the capture–recapture model (Boulanger and McLellan 2001, Boulanger et al. 2004,
Dice 1938, Karanth and Nichols 1998). These models also do not formally account
Northeastern Naturalist
146
C.A. Bozarth, B. Gardner, L.L. Rockwood, and J.E. Maldonado
2015 Vol. 22, No. 1
for the detection of heterogeneity that results from the lower likelihood of detecting
animals that live on the edge of the study site.
To address these concerns, we used a spatially explicit capture–recapture model
that incorporated detection-location information with detection history to eliminate
the need for post hoc buffering, and used a Bayesian framework to effectively deal
with a small sample size. This class of models has been successfully applied to
genotypes derived from fecal DNA data (e.g., Moore and Vigilant 2014), as well as
genotypes derived from hair samples (e.g., Gardner et al. 2010b, Kéry et al. 2011,
Russell et al. 2012), data collected from camera traps (e.g., Gardner et al. 2010a,
Reppucci et al. 2011, Royle et al. 2011), and a variety of other sources. The objective
of this study was to describe demographic parameters for a newly colonized
population of Coyotes and to use this new class of models to provide the first estimates
of Coyote density in the mid-Atlantic region.
Field-site Description
We conducted this study at Marine Corps Base Quantico (MCBQ), located 35
miles south of Washington, DC, in Virginia, and spanning 243 km2 over 3 counties
(Fauquier, Prince William, and Stafford). The base was established in 1917 and
was expanded during World War II to its current size. Though MCBQ was an active
military reservation, less than 30% of its holdings were used for strictly military operations.
The majority of the land was used for a suite of purposes including forest
and wildlife management, potable water production, waste disposal, and outdoor
recreation (Marine Corps Base Quantico 1996). In the face of burgeoning suburban
development that now completely surrounds the base, MCBQ has become a de
facto wildlife preserve, hosting a wide variety of plant and animal species (C.A.
Bozarth, pers. observ.). The study site was composed of second-growth deciduous
forest, open fields, lakes, streams, and human development. A 4-lane highway
(I-95) divides the base into western and eastern sides, with a secondary road connecting
the sides and running underneath the highway. Coyotes were first observed
on MCQB in 1997 (T. Stamps, MCBQ Natural Resources and Environmental Affairs,
pers. comm.). Coyotes are known to adjust their behavior and habitat use to
avoid human activity (Gehrt et al. 2009, Riley et al. 2003, Tigas et al. 2002), and
thus, the Coyote population at MCBQ should be more insular than in a landscape
with a patchy matrix of developed and non-developed areas.
Methods
Sample collection
We collected 331 carnivore scats at MCBQ during November 2006–October
2008. We systematically removed scat each month along the same eight 500-m
transects on roads dispersed throughout the base and then collected scat 6–10 days
later to ensure the freshness of the scat samples for molecular analyses. We marked
the location of each scat collection on a GIS map using Google Earth v5.0 (Keyhole,
Inc. 2009). Although transects were non-random due to the restrictions of
Northeastern Naturalist Vol. 22, No. 1
C.A. Bozarth, B. Gardner, L.L. Rockwood, and J.E. Maldonado
2015
147
ongoing military training at the site, randomly generated locations would have been
ineffective because carnivores often deposit scats on roadways (Macdonald 1980),
and humans are inefficient at locating scat in vegetation (Smith et al. 2001, 2003).
We also obtained tissue samples from 7 Coyotes killed by hunters on the base. We
included these samples as amplification controls and to measure the power of our
methods to distinguish individual animals when screening microsatellite loci (see
below), but did not use them in the spatially explicit population-abundance model
because we did not have precise locality data for them. We stored collected scats
and tissues in zip-locked plastic bags in the field and within hours of collection
moved them to freezers set at -4 °C.
Genetic data collection
We extracted DNA from scats using QIAamp DNA Stool Mini Kits and from
tissues using DNeasy DNA Extraction Kits (Qiagen Inc., Valencia, CA). To minimize
the risk of contaminating stock DNA with post-PCR products, we conducted
DNA isolation in a dedicated lab with a separate air-handling system from the
area where PCR amplifications were conducted. We also used aerosol-barrier tips
for all pre-PCR procedures to eliminate sample-to-sample contamination and used
negative controls in the DNA extraction and PCR amplification steps to control
for contamination.
Scat of sympatric carnivore species may not be readily identifiable by visual
examination (Davison et al. 2002); thus,we identified species by amplifying a short
section of the control region that is variable in length for each of the potential canid
species (Bozarth et al. 2010). We reliably differentiated scat deposited by Coyote,
Urocyon cinereoargenteus (Schreber) (Gray Fox), and Vulpes vulpes L. (Red Fox).
We removed samples that did not amplify from the data set.
We verified individual identities of animals detected from scat using up to 6
highly variable tetranucleotide microsatellite-loci (not all 6 loci amplified for all
samples) and used tissue samples as “known individual” controls. We used primers
FH2001, FH2096, FH2137, FH2140, FH2159, and FH2235 obtained from
the canine genome map (Francisco et al. 1996) and previously adapted for use in
population genetic studies of Coyotes by Prugh et al. (2005). We performed PCR
amplification in a 25-μL-reaction volume containing: 5 μL of template DNA (directly
from kit extraction); 0.5 μM of each FAM, HEX, or TET-labeled forward and
unlabeled reverse primer; 1x PCR Buffer II (Applied Biosystems, Foster City, CA);
2 mM MgCl2; 1 unit of Amplitaq Gold™ polymerase (Applied Biosystems); 0.008
mg/mL bovine serum albumin (BSA, New England Biolabs, Ipswich, MA); and 0.2
mM deoxynucleoside triphosphate. We employed negative and positive controls
for each PCR reaction. We amplified DNA in PTC-100 and PTC-200 thermocyclers
(MJ Research, Waltham, MA) using the following program: initial denaturation at
95 °C for 10 min; 45 cycles of 1 min at 95 °C, 1 min at 58 °C, and 1 min at 72 °C;
and final extension at 72 °C for 10 min. We separated and detected PCR products by
capillary electrophoresis on an ABI 3130xl Genetic Analyzer (Applied Biosystems)
and an ABI 3100 Genetic Analyzer (Applied Biosystems). We analyzed gels with
Northeastern Naturalist
148
C.A. Bozarth, B. Gardner, L.L. Rockwood, and J.E. Maldonado
2015 Vol. 22, No. 1
GeneMapper Analysis Software version 4.0 (Applied Biosystems) and typed all 7
Coyote tissue samples at these same loci.
We assigned sex to individuals using amplification and restriction-enzyme digestion
of sex-chromosomal zinc-finger genes, following protocols designed for
Kit Foxes and other canids in Ortega et al. (2004). Using this method, we amplified
a short (195-bp) fragment of the zinc-finger protein genes (Zfx and Zfy) that contains
a TaqI digestion site unique to the Zfy gene. When the TaqI enzyme digests
these fragments, products show a single band for females and a double band for
males. We separated and detected PCR products by capillary electrophoresis on
an ABI 3130xl Genetic Analyzer (Applied Biosystems). We analyzed gels with
GeneMapper Analysis Software version 4.0 (Applied Biosystems).
Analysis of microsatellite DNA
We subjected each DNA extract to independent PCR amplifications for each locus
at least 3 times for heterozygotes and 5 times for homozygotes as recommended
by Taberlet et al. (1996) for non-invasive samples. After each sample was successfully
typed at all 6 loci, we determined the reliability of each observed multilocus
score using the program Reliotype, which assesses the reliability of an observed
multilocus genotype using a maximum-likelihood approach for minimizing genotyping
errors (Miller et al. 2002). Due to the low-quality DNA often present in scat
samples (Taberlet et al. 1996), not all sample extracts amplified at all 6 loci. For
each of the samples with incomplete data, we compared known loci (verified by at
least 3 independent amplifications) to complete genotypes by eye to conservatively
assign new genotypes to these samples when possible. To estimate the probability
that individuals can be differentiated by these 6 loci, we calculated the unbiased
probability of observing identical multilocus genotyopes between 2 individuals
sampled from the population for unrelated individuals (PID-UNBIAS) and for siblings
(PID-SIBS) using the program GIMLET (Valière 2002). We also confirmed that the
genotypes were unique at all 6 loci for our 7 Coyote tissue sam ples.
We used GENEPOP version 4.0 (Raymond and Rousset 1995) to calculate allelic
diversity and to test for departure from Hardy-Weinberg and genotypic linkage
equilibrium. To control for false positives that may arise in multiple comparisons,
we employed a sequential Bonferroni correction (Holm 1979). We calculated observed
heterozygosity (HO) and expected heterozygosity (HE) for each locus and for
the population using CERVUS version 3.0 (Marshall et al. 1998).
To investigate the colonization history of the Coyote population, we evaluated
the relatedness of sampled individuals. A high level of relatedness between
individuals may indicate colonization by a single group of related individuals, as
opposed to colonization by multiple unrelated individuals. To estimate pairwise
relatedness (R), we used KINSHIP version 1.3.1 (Goodnight and Queller 1999) and
the method described by Queller and Goodnight (1989). We also used KINSHIP
to test the likelihood that individuals were related at the level of full-siblings. The
program calculates the likelihood of the primary hypothesis (R = 0.5) and the null
hypothesis (R = 0) for each pair of individuals. To calculate significance, KINSHIP
Northeastern Naturalist Vol. 22, No. 1
C.A. Bozarth, B. Gardner, L.L. Rockwood, and J.E. Maldonado
2015
149
runs a simulation that generates pairs of individuals using the primary hypothesis
and allele frequencies of the data set to determine the ratio needed to reject the null
hypothesis at various significance levels.
Due to their great dispersal ability, Coyote populations have been shown to
exhibit low levels of genetic structure (Lehman and Wayne 1991, Roy et al. 1994).
However, because this population was recently colonized and the habitat in the
area is heavily fragmented and separated by roads and a 6-lane highway with heavy
traffic 24 hrs/day, there was a potential for restricted gene flow within the study
area. To test for signatures of population genetic structure, we used the Bayesian
model-based approach implemented in STRUCTURE v2.3.2 (Pritchard et al. 2000).
STRUCTURE uses discontinuities in multilocus allele profiles detected in terms of
Hardy-Weinberg equilibrium and linkage disequilibrium to assign each genotype
to a genetic cluster. Because there are no a priori assumptions about population
assignments, STRUCTURE can provide an unbiased indicator of geographical patterns
of population structure. Because we expected high levels of gene flow within
the study site, we used the population admixture model, which assigns genotypes
probabilistically to clusters and thereby allows individuals to have mixed ancestry
from different clusters. We ran 2 sets of simulations: 1 with alleles independent
and 1 with alleles correlated as recommended by Pritchard et al. (2010). For each
of these 2 trials, the user specifies the number of clusters (K). Because we assumed
high levels of gene flow, we expected to find the best support for K = 1 (panmixia).
We ran each simulation (for K = 1 … 6) 5 times (100,000 iterations burn-in and
then 100,000 iterations MCMC). We used the program Structure Harvester v0.56.4
(Earl 2009) to identify the most likely K. We used the program CLUMPP v1.1.2
(Jakobsson and Rosenberg 2007) to visualize the mean of multiple STRUCTURE
runs for the optimal K.
Spatially explicit model
To estimate the density of Coyotes and the population size at the study site using
fecal DNA, we applied a spatial capture–recapture model (Borchers and Efford
2008, Gardner et al. 2009, Royle et al. 2009). The model addresses the movement
of individuals by assuming that each individual has an activity center and that the
probability of capture is a monotonic function of the distance from the activity center
to a capture location (see Royle et al. 2014 for more details). We recorded the
latitude and longitude of the centroid of each transect and used this as the “trap” location.
For this model, we then summed the encounters across the repeat detections
for each of the 2 years of study. We combined the data for both years to estimate
the baseline encounter rate and σ (the scaling parameter for the distance function),
but we estimated population size for each sampling period separately (November
2006–October 2007 and November 2007–October 2008) to account for possible
population-size fluctuations.
The model can be formulated as a generalized linear mixed model. To begin, we
assumed that each individual i in the population had an activity center at a fixed
point, si = (s1i, s2i) for i = 1, 2, … n individuals and these activity centers were
uniformly distributed across some region S. We denoted the transect locations as
Northeastern Naturalist
150
C.A. Bozarth, B. Gardner, L.L. Rockwood, and J.E. Maldonado
2015 Vol. 22, No. 1
xj = (x1j, x2j) for j = 1, 2, … J transects. Next, we defined the encounter history yijkt,
i.e., the number of times an individual i was captured in transect j during a sampling
occasion k in year t, as a Poisson random variable with mean λij such that:
yijkt ~ Poisson (λij).
We used a Poisson distribution to allow for the potential of multiple recaptures of
an individual at a sampling location during any sampling occasion. To model the
relationship between an individual i’s activity center and the transect j, we used
the half-normal detection function. This yields a log-linear model of the form
used by Royle et al. (2009) and results in the following log-link formulation:
log (λij) = log (λ0) + (1 / 2σ2 )dij
2,
where dij
2= ||si xj||2 is the squared distance from individual i’s activity center to
transect j, s is the scaling parameter for the distance function, and λ0 is the baseline
encounter rate, defined as the expected number of captures at a sampling location
during a sampling occasion given that an individual’s activity center is located
precisely at that sampling location.
The activity centers, si, are not observed in this formulation of the model, and
thus, dij
2 is a latent random effect. To carry out inference on N, the number of
activity centers (si) in the state-space S, we assumed that the si’s are distributed
uniformly over some prescribed region S, an arbitrarily large polygon containing
the sampling locations. Because N is unknown, we used a technique called data
augmentation (Royle et al. 2007), which effectively creates a zero-inflated version
of the data set. Based on this formulation of the model, density is a derived
parameter estimated as N/|S|, where N is the estimated number of activity centers
(si) in the state-space S |S| is the area of S. The state-space and data augmentation
are statistical tools to estimate abundance; however, caution should be taken in
making inferences outside the surveyed area if conditions (e.g., habitat) in the
state-space are not consistent with those observed in the surveyed area. For this
analysis, we specified S as a rectangle centered on the study site with an area of
1452 km2. We augmented the data by adding 97 more potential individuals with
all zero encounter histories. Ro more information on choice of state-space and
data augmentation, see Chapter 5 of Royle et al. (2014). We used a Bayesian
framework for analysis of the model. We checked the output to ensure the chains
converged by visual inspection and by checking that all Ȓ values were less than
1.1 (Gelman and Hill 2007). We implemented the model in the freely available
software package WinBUGS (Gilks et al. 1994). Posterior parameter estimates
are based on 3 chains run for 15,000 iterations after a burn-in period of 5000 and
iterations thinned by 3. In this model, transient individuals are assumed to occur
randomly in the study.
We also ran program GIMLET (Valière 2002) with both 1000 and 10,000 iterations
using the Kohn et al. (1999), Chessel (Valière 2002), and Eggert et al. (2003)
methods to verify that there were not enough recaptures to estimate population size
using rarefaction.
Northeastern Naturalist Vol. 22, No. 1
C.A. Bozarth, B. Gardner, L.L. Rockwood, and J.E. Maldonado
2015
151
Results
We successfully identified 77 Coyotes, 62 Gray Fox, and 59 Red Fo x out of the
331 scats collected. We did not identify scat deposited by either Canus lupus familiaris
L. (Domestic Dog) or Gray Wolf. Coyotes in Northern Virginia are known
to have hybridized with Canis lycaon Say (Eastern Wolf; Bozarth et al. 2011); the
hybrids are known as Coywolves. Our method of species identification does not
differentiate between Coyote and Eastern Wolf, ensuring that all Coywolves are
appropriately included in the analysis. Of the Coyote samples, 41 met our criteria
for identifying microsatellite alleles and could confidently be assigned a genotype
(53% success rate). We found 30 unique genotypes, 23 of which were detected
from scat samples and 7 from tissue samples from the individuals killed by hunters.
Two genotypes, represented by only 1 scat sample each, were unique based on
4 and 5 loci, respectively, and we confirmed each locus with multiple successful
amplifications. All other individual identifications were based on the full 6 loci.
All 6 microsatellite loci were polymorphic in this population, with an average of
8 alleles/locus (range = 6–11 alleles/locus). We found locus FH2137 to be significantly
out of Hardy-Weinberg equilibrium after sequential Bonferroni correction
and locus FH2159 approaching significance (P = 0.0115) after Bonferroni. Observed
and expected heterozygosity for these 2 loci ranged from 0.533–0.933 and
0.684–0.854, respectively, with locus FH2159, but not locus FH2137, showing
significant homozygote excess after Bonferroni correction (P = 0.003 and 0.049,
respectively). We found departures from linkage equilibrium at 5 of 15 comparisons
(4 of which included locus FH2159). Average HO was 0.756 and HE was 0.798. We
found an estimated overall PID-UNBIAS of 5.217e-08 and an estimated overall PID-SIBS
of 3.197e-03. None of the genotypes recovered from scat samples matched the
genotypes from the 7 tissue samples, and none of the 7 tissues known to come from
different animals had identical genotypes, corroborating that we had high power to
distinguish individual animals using our loci. We found 18 males, 11 females, and
1 genotype for which sex could not be determined, despite multiple replicates for
each PCR.
The estimated relatedness (R) between all pairs of individuals ranged from
-0.470 to 0.912, with the average relatedness for the entire population at 0.001.
When tested against the primary hypothesis of R = 0.50 (relatedness coefficient for
full siblings), only 31 out of 435 pairwise comparisons were significant, indicating
that most sampled individuals were not closely related.
For the STRUCTURE model with alleles correlated, alpha converged but with
high variability, and the plot of delta K did not peak (as expected for resolved data
sets). For the model with alleles independent, alpha converged, and the results
were more discernable. The plot of mean log likelihood indicated K = 3 as the
best fit for the data. The plot of delta K produced a clear peak at K = 3. In examining
the Q-plots for each of the 5 replicate runs of K = 3, we found that 4 of the
5 clearly divided individuals into 3 clusters and 1 did not. We used the program
CLUMPP to find the mean of the K = 3 outputs (including the replicate of K = 3
that did not divide individuals as clearly into 3 clusters; excluding it produced
Northeastern Naturalist
152
C.A. Bozarth, B. Gardner, L.L. Rockwood, and J.E. Maldonado
2015 Vol. 22, No. 1
similar results) and could distinguish the 3 clusters (Fig. 1). To visualize population
structure at the study site, we mapped the proportion of membership in each
of the K = 3 clusters for each of the 8 transects and then for the west and east
sections of the base, as divided by I-95. Division by transects did not produce
any discernible pattern, but division by I-95 indicated some structure, though we
collected few samples east of I-95. Two of the genotypes (19 and 17) that were
assigned to cluster 2 (0.827 and 0.845 membership respectively) were only detected
east of I-95. A third genotype (30) only detected east of I-95 had 0.433
Figure 1. (a) Mean of 5 replicates of genetic assignment results for Coyotes at MCBQ for
K = 3 from the program STRUCTURE as averaged by the program CLUMPP. Genetic population
clusters are coded with different shades of gray. The fraction of each color for each
individual represents the probability of assignment to that cluster. (b) Magnitude of ΔK (rate
of change in the log probability) and lnP(K) (posterior probability of the data) as a function
of K (populations) detected 3 genetic clusters in the sampled population.
Northeastern Naturalist Vol. 22, No. 1
C.A. Bozarth, B. Gardner, L.L. Rockwood, and J.E. Maldonado
2015
153
membership in cluster 2. The only other individual that had high assignment to
cluster 2 (genotype 29) was found west of I-95. More intensive sampling east of
I-95 is needed to strengthen these tentative results.
Genetic results showed that the 23 Coyote individuals made 41 visits to the 8
transects during the 2-year survey. During the survey, encounters at each transect
varied from 1 to 12, with 12 individuals detected only once. For the two different
study periods, we estimated the posterior mean number of activity centers (N̑ ) as
58.81 and 75.88, respectively, in the state-space (S = 1452 km2) and the density
(D̂ ) at 0.04 (95%BCI: 0.02, 0.07) and 0.05 (95%BCI: 0.03, 0.08) individuals/km2
in the two periods, respectively. We used the area of the base (243 km2) to estimate
the number of individuals with activity centers on the base at 9.96 individuals in
the first year and 12.69 individuals the second year (mean = 11.3 individuals). The
posterior mean estimate for σ, the scaling parameter for the distance function, was
2.60 with a 95% posterior interval of (1.92, 3.58). Based on the posterior mean estimate
of σ, we estimated the mean home-range radius to be 6.33 km by assuming a
bivariate normal model for movement. The posterior mean for λ was estimated at
0.11 with a 95% posterior interval of (0.05, 0.21). λ is the baseline encounter rate
under the Poisson encounter model used here, which resulted in an estimated detection
probability of 0.10. The combination of low detection and larger σ suggests
that Coyotes utilizing MCBQ have activity centers located not just within the base
but also in the surrounding areas. This pattern is also seen in the posterior densities
of activity centers for captured and uncaptured individuals, which indicates spatial
heterogeneity in Coyote density (Fig. 2). The posterior summaries of model parameters
are summarized in Table 1.
None of the rarefaction methods in program GIMLET produced a curve with an
asymptote.
Discussion
We found high levels of polymorphism and heterozygosity in this newly colonized
population of Coyotes. Given the precautions we took for multiple replicate
PCR reactions, the departure from Hardy-Weinberg equilibrium for locus FH2137
as well as the departure from linkage equilibrium and homozygote excess for
locus FH2159 were most likely due to small sample size and not allelic dropout.
Table 1. Posterior summaries of model parameters for this study of Coyotes at Marine Corps Base
Quantico, where 23 individual Coyotes were observed during 2 years. We define N1and N2 as the
number of estimated activity centers in the state-space S (rectangular area of 1452 km2 around the
study area used for analyses) during years 1 and 2 respectively. The baseline encounter rate under the
Poisson encounter model is λ0 and the scaling parameter for the distance function is σ.
Mean SD 2.5% Median 97.5%
λ0 0.11 0.04 0.05 0.11 0.21
σ 2.60 0.43 1.92 2.55 3.58
N1 58.81 17.832 29.00 47.00 99.00
N2 75.88 19.12 42.00 62.00 114.00
Northeastern Naturalist
154
C.A. Bozarth, B. Gardner, L.L. Rockwood, and J.E. Maldonado
2015 Vol. 22, No. 1
Additionally, all 6 loci have previously been used in a population genetic study
on Coyotes and were found to have insignificant error rates (P < 0.01), taking into
account both allelic dropout and false allele rates (Prugh et al. 2005). The average
HO of 0.756 is similar to values reported for Coyote populations in other regions
(0.69–0.74; Prugh et al. 2005, Riley et al. 2006, Sacks et al. 2005). The male to
female sex ratio was slightly skewed at 1.6:1. This finding could be the result of
the small sample size (30 individuals over a 2-year period) or differences in the
detection of the sexes; our skewed sex ratio is probably not due to the detection
of transient individuals because males and females are equally common dispersers
(Bekoff 1977).
Pairwise relatedness was low between most pairs of individuals (R = 0.001).
Because the program KINSHIP bases relatedness values on allele frequencies
Figure 2. Map of the posterior mean density of activity centers for Coyotes across the state
space for year 1 of sampling, specifically, E[N1(b)|data], where N1(b) is the estimated number
of activity centers located in pixel b. MCBQ is outlined in black, black asterisks represent
the location of the center of the sampled transects, black lines show Interstate 95 and
other highways, and dark gray polygons represent major waterways. The map is displayed
in universal transverse mercator (UTM) Zone 18 north coordinates with 1 km x 1 km cells.
Northeastern Naturalist Vol. 22, No. 1
C.A. Bozarth, B. Gardner, L.L. Rockwood, and J.E. Maldonado
2015
155
given in the data instead of a population of random individuals, relatedness values
may be biased low. Thus, we can conservatively state that the sampled population
was composed of unrelated individuals, which may indicate multiple colonization
events from diverse geographical regions.
The STRUCTURE assignment tests determined that 3 population clusters best
fit the data. We expected to find panmixia (K = 1) because of the high gene flow
and previously reported weak genetic structure in Coyote populations (Lehman
and Wayne 1991) and in other canid species (Gray Wolf: Vilà et al. 1999; Vulpes
lagopus (L.) [Arctic Fox]: Dalen et al. 2005). The detection of 3 distinct population
clusters may support the fact that this newly colonized population of Coyotes
was the result of multiple sources of colonization from several family groups, as
opposed to a single colonization event by members of 1 closely related group of
individuals. In addition, a previous genetic study using mitochondrial DNA from
the same individuals in this population also detected 5 different matrilines, all of
which have been previously reported in diverse surrounding geographic localities,
and is further evidence of multiple sources of colonization from unrelated individuals
(Bozarth et al. 2011). The apparent genetic structure caused by the division of
the base by I-95 could be due to our small sample size east of the highway, although
previous studies have found that a highway can be a genetic (but not physical) barrier
to Coyote movement in southern California (Riley et al. 2006). In addition,
results from the mtDNA analysis in Bozarth et al. (2011) show the presence of 5
haplotypes at MCBQ, with all occurring west of the highway, but 1 haplotype occurring
only east of the highway. More extensive sampling on both sides of the
highway may strengthen the preliminary evidence of structure found here.
The population-density estimates at MCBQ (mean = 0.047/km2) are comparable
to estimates on urbanized Cape Cod, MA (0.06–0.15 individuals/km2; Way et al.
2002). Our slightly lower estimate may be due to lower availability of food, the
recentness of the colonization, or violation of model assumptions. Coyote density
is strongly correlated with the abundance of potential prey (e.g., Clark 1972, Mills
and Knowlton 1991, O’Donoghue et al. 1998, Prugh et al. 2005, Rose and Polis
1998). At the study site, however, the density of small mammals, anthropogenic
food sources (including road kill), and other prey items appear to be abundant (Robinson
2005). In addition, Coyotes, as generalist predators, make use of a variety of
food sources on the base, including Odocoileus virginianus Zimmermann (White-
Tailed Deer), fruits, insects (including crickets and beetles), and birds (Robinson
2005). Because the density estimate is lower than, but still similar to, estimates on
Cape Cod, the effect of food availability may not be strong enough to tease apart
the slight differences in density estimates. Other examples of low Coyote density
estimates occur during prewhelping, when densities are expected to be biased low
because of reduced detectability (0.01 individuals/km2; Springer and Wenger 1981)
and during winter surveys in harsh climates where Coyote densities are expected to
be genuinely low due to mortality (0.05 individuals/km2; Brock 1992). A slightly
low population-density estimate may also be due to the recentness of the Coyote
colonization (see Parker [1995:81] for a discussion of fluctuating population
Northeastern Naturalist
156
C.A. Bozarth, B. Gardner, L.L. Rockwood, and J.E. Maldonado
2015 Vol. 22, No. 1
densities in newly colonized populations). Though density estimates at our site
were consistent over a 2-year period, density was slightly higher the second year.
Coyotes have only been recorded at MCBQ since 1997, making this a very recent
colonization. The high number of individuals detected by genotyping from scats
and the high haplotype diversity (Bozarth et al. 2011) of this population also indicate
a population still in flux. Finally, our density estimate may be biased due to the
small number of sampling transects and the wide spacing, which resulted in a low
number of captures and recaptures. Of all individuals identified, only 3 were detected
on more than one transect, none were detected at more than 2 transects, and
13 were detected only once. If transects had been located closer to one another, we
would have expected more recaptures, and this is an important study-design issue
for future studies to consider when placing transects or trap locations (see Sollmann
et al. 2012, Sun et al. 2014). In order to fit the model to this small amount of data,
we had to assume a demographically closed population over the duration of each
sampling year. This assumption was most likely violated and may also have caused
bias in our estimates of density.
The overall picture of Coyote population density in the literature is one of incredible
variation, due to a variety of factors. Estimates of density range from 2.0
individuals/km2 in southern Texas (Windberg and Knowlton 1988) to the smallest
density estimates discussed above. Kays et al. (2008) conducted a large-scale scat
survey of Coyotes in the Adirondacks region of northern New York and found that
the number of scats captured was directly proportional to the number of individuals
detected. There is much variation in density estimates, which suggests that scat
surveys can be a useful tool for providing unbiased density estimates.
With the half-normal detection function, we can convert the scale parameter
(σ) to a home-range radius (6.33 km) of a circle. While it is understood that home
ranges are never exactly circular in nature (Samuel and Garton 1985, Smith 1983),
this fact does not imply that using a circular detection function is inadequate or unrealistic.
This assumption is necessary for the model, and recent studies show that
selective space usage, which would violate this assumption of home-range shape,
does not bias estimates of population size in SCR models (Efford 2014). Converting
the home-range radius (6.33 km) to the area of the circle using the formula area
= π (radius)2 yields a rather large estimate of 125.82 km2. Home-range sizes for
Coyotes are highly variable, ranging from 4.1 km2 in northeastern Kansas (Kamler
and Gipson 2000) to 219.7 km2 for transient Coyotes in Southern British Columbia
(Atkinson and Shackleton 1991), though most reported home-range sizes are
between 12 and 50 km2 (e.g., Gese et al. 1988, Grinder and Krausman 2001, Harrison
et al. 1989, Holzman et al. 1992, Major and Sherburne 1987, Way et al. 2002,
Windberg et al. 1997). In our study, the paucity of recaptures (41 total captures
for 23 individuals), the low number of captures, resultant scarcity of data, and the
potential for transients to be included may have inflated this h igh home-range size
estimate (hence the wide confidence bounds on σ; Table 1).
The map of the posterior density of activity centers (Fig. 2) highlights the need
for dealing with spatial heterogeneity in a capture-recapture model. Coyote activity
Northeastern Naturalist Vol. 22, No. 1
C.A. Bozarth, B. Gardner, L.L. Rockwood, and J.E. Maldonado
2015
157
is centered on one area of the study site, which coincides with the presence of an old
landfill site. The landfill is covered and now exists as an open area dominated by tall
grass, with planted Pinus (pine) trees and natural second-growth forest surrounding
it, making this area an ideal habitat for Coyotes. The presence of a perennial pond
may also attract Coyotes as well as potential prey species. The spatially explicit
model we used directly addresses this spatial heterogeneity by using the location
of individuals in relation to the captures. These hotspots of activity can be used
to better understand Coyote habitat preferences in a heterogeneous landscape and
inform population management.
Using noninvasive molecular techniques, we characterized the genetic variability,
population relatedness, and population structure of a newly colonized Coyote
population. We predict that population density and genetic structuring across the
highway will increase in the future as more Coyotes exploit the abundant food
sources and establish territories. Additionally, we have used a new class of spatially
explicit capture–recapture models that effectively deal with violations of
closure and small sample size to estimate the population density, population size,
home-range radius, and detection probability of these Coyotes. By sharing some
parameters across years, we were able to make inferences despite the small sample
sizes in each year. This population genetic study of Coyotes in the mid-Atlantic
region focused on combining this new class of spatial capture–recapture models
with DNA data collected from scat. Molecular studies using fecal DNA and spatially
explicit capture–recapture models could also be used to estimate these key
demographic parameters for other elusive animals.
Acknowledgments
Funding was provided by Naval Facilities Engineering Command Washington Cooperative
Agreement N40080-08-LTC-0001 for Coyote Population Study at MCB Quantico
and the Smithsonian Conservation Biology Institute’s Center for Conservation and Evolutionary
Genetics. We thank T. Stamps, C. Himmelberger, N. McInerney, T. Wilbert,
K. Robinson, C. Garcia, L. Lakeman, M. Tsuchiya, and C. Edwards for samples, assistance,
and guidance.
Literature Cited
Archie, E.A., J.A. Hollister-Smith, J.H. Poole, P.C. Lee, C.J. Moss, J.E. Maldonado, R.C.
Fleischer, and S.C. Alberts. 2007. Behavioral inbreeding avoidance in wild African Elephants.
Molecular Ecology 16:4138–4148.
Archie, E.A., J.E. Maldonado, J.A. Hollister-Smith, J.H. Poole, C.J. Moss, R.C. Fleischer,
and S.C. Alberts. 2008. Fine-scale population genetic structure in a fission–fusion society.
Molecular Ecology 17:2666–2679.
Atkinson, K.T., and D.M. Shackleton. 1991. Coyote, Canis latrans, ecology in a rural–urban
environment. The Canadian Field-Naturalist 105:49–54.
Battiata, M. 2006. Meet the neighbors. The Washington Post Magazine.16 April 2006.
Bekoff, M. 1977. Canis latrans. Mammalian Species 79:1–9.
Bellemain, E., J.E. Swenson, D. Tallmon, S. Brunberg, and P. Taberlet. 2005. Estimating
population size of elusive animals with DNA from hunter-collected feces: Four methods
for Brown Bears. Conservation Biology 19:150–161.
Northeastern Naturalist
158
C.A. Bozarth, B. Gardner, L.L. Rockwood, and J.E. Maldonado
2015 Vol. 22, No. 1
Borchers, D.L., and M.G. Efford. 2008. Spatially explicit maximum likelihood methods for
capture–recapture studies. Biometrics 64:377–385.
Boulanger, J., and B. McLellan. 2001. Closure violation in DNA-based mark–recapture
estimation of Grizzly Bear populations. Canadian Journal of Zoology 79:642–651.
Boulanger, J., G. Stenhouse, and R. Munro. 2004. Sources of heterogeneity bias when DNA
mark–recapture sampling methods are applied to Grizzly Bear (Ursus arctos) populations.
Journal of Mammalogy 85:619–624.
Bozarth, C.A., Y.R. Alva-Campbell, K. Ralls, T.R. Henry, D.A. Smith, M.F. Westphal, and
J.E. Maldonado. 2010. An efficient noninvasive method for discriminating among feces
of sympatric North American canids. Conservation Genetics Resources 2:173–175.
Bozarth, C.A., F. Hailer, L.L. Rockwood, C.W. Edwards, J.E. Maldonado. 2011. Coyote
colonization of northern Virginia and admixture with Great Lakes Wolves. Journal of
Mammalogy 92(5):1070–1080.
Brocke, R.H. 1992. A taste for venison. Natural History. May:50–51.
Clark, F.W. 1972. Influence of Jackrabbit density on Coyote population change. Journal of
Wildlife Management 36:343–356.
Dalen, L., E. Fuglei, P. Hersteinsson, C.M.O. Kapel, J.D. Roth, G. Samelius, M. Tennerfeldt,
and A. Angerbjorn. 2005. Population history and genetic structure of a circumpolar
species: The Arctic Fox. Journal of the Linnean Society 84:79–89.
Davison, A., J.D.S. Birks, R.C. Brookes, T.C. Braithwaite, and J.E. Messenger. 2002. On
the origin of feces: Morphological versus molecular methods for surveying rare carnivores
from their scats. Journal of Zoology 257:141–143.
Dice, L. 1938. Some census methods for mammals. Journal of Wildlife Management
2:119–130.
Earl, D.A. 2009. Structure Harvester v0.3. Available online at http://users.soe.ucsc.
edu/~dearl/software/struct_harvest/. Accessed 21 June 2010.
Efford, M.G. 2004. Density estimation in live-trapping studies. Oikos 106:598–610.
Efford, M.G. 2014. Bias from heterogeneous usage of space in spatially explicit capture–
recapture analyses. Methods in Ecology and Evolution 5:599–602.
Eggert, L., J. Eggert, and D. Woodruff. 2003. Estimating population sizes for elusive
animals: The forest elephants of Kakum National Park, Ghana. Molecular Ecology
12:1389–1402.
Flagstad, O., E. Hedmark, A. Landa, A. Broseth, J. Persson, R. Andersen, P. Segerstrom,
and H. Ellegren. 2004. Colonization history and noninvasive monitoring of a reestablished
Wolverine population. Conservation Biology 18:676–688.
Francisco, L.V., A.A. Langston, C.S. Mellersh, C.L. Neal, and E.A. Ostrander. 1996. A class
of highly polymorphic tetranucleotide repeats for canine genetic mapping. Mammalian
Genome 7:359–362.
Gardner, B., J.A. Royle, and M.T. Wegan. 2009. Hierarchical models for estimating density
from DNA mark–recapture studies. Ecology 90:1106–1115.
Gardner, B., J. Reppucci, M. Lucherini, and J.A. Royle. 2010a. Spatially explicit inference
for open populations: Estimating demographic parameters from camera-trap studies.
Ecology 91:3376–3383.
Gardner, B., J.A. Royle, M.T. Wegan, R.E. Rainbolt, and P.D. Curtis. 2010b. Estimating
Black Bear density using DNA data from hair snares. Journal of Wildlife Management
74:318–325.
Gehrt, S.D., and S. Prange. 2007. Interference competition between Coyotes and Raccoons:
A test of the mesopredator-release hypothesis. Behavioral Ecology 18:204–214.
Northeastern Naturalist Vol. 22, No. 1
C.A. Bozarth, B. Gardner, L.L. Rockwood, and J.E. Maldonado
2015
159
Gehrt, S.D., C. Anchor, and L.A. White. 2009. Home range and landscape use of Coyotes in a
metropolitan landscape: Conflict or coexistence? Journal of Mammalogy 90:1045–1057.
Gelman, A., and J. Hill. 2007. Data Analysis Using Regression and Multilevel Hierarchical
Models. Cambridge University Press, New York, NY. 648 pp.
George Mason University Center for Regional Analysis (GMUCRA). 2010. Update from
the 2010 census. Available online at http://cra.gmu.edu/pdfs/researach_reports/recent_
reports/Population_Change_in_Northern_Virginia.pdf. Accessed 11 February 2015.
Gese, E.M., O.J. Rongstad, and W.R. Mytton. 1988. Home range and habitat use of Coyotes
in southeastern Colorado. Journal of Wildlife Management 52:640–646.
Gilks, W.R., A. Thomas, and D.J. Spiegelhalter. 1994. A language and program for complex
Bayesian modelling. The Statistician 43:169–177.
Goodnight, K.F., and D.C. Queller. 1999. Computer software for performing likelihood
tests of pedigree relationship using genetic markers. Molecular Ecology 8:1231–1234.
Grinder, M.I., and P.R. Krausman. 2001. Home range, habitat use, and nocturnal activity of
Coyotes in an urban environment. Journal of Wildlife Management 65:887–898.
Hailer, F., and J.A. Leonard. 2008. Hybridization among three native North American Canis
species in a region of natural sympatry. PLoS ONE 3: e3333.
Harrison, D.J., J.A. Bissonette, and J.A. Sherburne. 1989. Spatial relationships between
Coyotes and Red Foxes in Eastern Maine. Journal of Wildlife Management 53:181–185.
Holm, S. 1979. A simple, sequential, rejective multiple-test procedure. Scandinavian Journal
of Statistics 6:65–70.
Holzman, S., M.J. Conroy, and J. Pickering. 1992. Home range, movements, and habitat
use of Coyotes in southcentral Georgia. Journal of Wildlife Management 56:139–146.
Jakobsson, M., and N. Rosenberg. 2007. CLUMPP: A cluster matching and permutation
program for dealing with label switching and multimodality in analysis of population
structure. Bioinformatics 23:1801–1806.
Kamler, J.F., and P.S. Gipson. 2000. Space and habitat use by resident and transient Coyotes.
Canadian Journal of Zoology 78:2106–2111.
Karanth, K.U., and J.D. Nichols. 1998. Estimation of Tiger densities in India using photographic
captures and recaptures. Ecology 79:2852–2862.
Kays, R.W., M.E. Gompper, and J.C. Ray. 2008. Landscape ecology of Eastern Coyotes
based on large-scale estimates of abundance. Ecological Applications 18:1014–1027.
Kéry, M., B. Gardner, T. Stoeckle, D. Weber, and J.A. Royle. 2011. Spatial capture–recapture
density estimation using DNA-sampled data for rare and elusive animals. Conservation
Biology 25:356–364.
Keyhole, Inc. 2009. Google Earth. Availableonline at http://earth.google.com. Accessed 15
April 2010.
Kohn, M.H., E.C. York, D.A. Kamradt, G. Haught, R.M. Sauvajot, and R.K. Wayne. 1999.
Estimating population size by genotyping feces. Proceedings of the Royal Society of
London B: Biological Sciences 266:657–663.
Lehman, N., and R.K. Wayne. 1991. Analysis of Coyote mitochondrial DNA genotype frequencies:
Estimation of the effective number of alleles. Genetics 128:405–416.
Lucchini, V., E. Fabbri, F. Marucco, S. Ricci, L. Boitani, and E. Randi. 2002. Noninvasive
molecular tracking of colonizing Wolf (Canis lupus) packs in the western Italian Alps.
Molecular Ecology 11:857–868.
Macdonald, D.W. 1980. Patterns of scent marking with urine and feces amongst carnivore
communities. Symposium of the Zoological Society of London 45:107–139.
Major, J.T., and J.A. Sherburne. 1987. Interspecific relationships of Coyotes, Bobcats, and
Red Foxes in western Maine. Journal of Wildlife Management 51:606–616.
Northeastern Naturalist
160
C.A. Bozarth, B. Gardner, L.L. Rockwood, and J.E. Maldonado
2015 Vol. 22, No. 1
Marine Corps Base Quantico. 1996. Fish and wildlife management plan. Section 3 of the
integrated natural resources management plan for the Marine Corps Base, Quantico, VA.
Marshall, T.C., J. Slate, L.E.B. Kruuk, and J.M. Pemberton. 1998. Statistical confidence
for likelihood-based paternity inference in natural populations. Molecular Ecology
7:639–655.
Mastro, L.L. 2011. Life history and ecology of Coyotes in the mid-Atlantic states: A summary
of the scientific literature. Southeastern Naturalist 10(4) :721–730.
Miller, C.R., P. Joyce, and L.P. Waits. 2002. Assessing allelic dropout and genotype reliability
using maximum likelihood. Genetics 160:357–366.
Mills, L.S., and F.F. Knowlton. 1991. Coyote space use in relation to prey abundance. Canadian
Journal of Zoology 69:1516–1521.
Miotto, R.A., F.P. Rodrigues, G. Ciocheti, and P.M. Galetti, Jr. 2007. Determination of the
minimum population size of Pumas (Puma concolor) through fecal DNA analysis in two
protected Cerrado areas in the Brazilian Southeast. Biotropica 39:647–654.
Moore, D.L., and L. Vigilant. 2014. A population estimate of Chimpanzees (Pan troglodytes
schweinfurthii) in the Ugalla region using standard and spatially explicit genetic capture–
recapture methods. American Journal of Primatology 76:335–346.
O’Donoghue, M., S. Boutin, C.J. Krebs, G. Zuleta, D.L. Murray, and E.J. Hofer. 1998.
Functional responses of Coyotes and Lynx to the Snowshoe Hare cycle. Ecology
79:1193–1208.
Ortega, J., M. del Rosario Franco, B.A. Adams, K. Ralls, and J.E. Maldonado. 2004. A reliable,
non-invasive method for sex determination in the endangered San Joaquin Kit Fox
(Vulpes macrotis mutica) and other canids. Conservation Genetics 5:715–718.
Parker, G. 1995. Eastern Coyote: The story of Its Success. Nimbus Publishing, Halifax,
N.S., Canada. 254 pp.
Pritchard, J.K., M. Stephens, and P. Donnelly. 2000. Inference of population structure using
multiplocus genotype data. Genetics 155:945–959.
Prugh, L.R., C.E. Ritland, S.M. Arthur, and C.J. Krebs. 2005. Monitoring Coyote population
dynamics by genotyping feces. Molecular Ecology 14:1585–1596.
Queller, D.C., and K.F. Goodnight. 1989. Estimating relatedness using genetic markers.
Evolution 43(2):258–275.
Raymond, M., and F. Rousset. 1995. GENEPOP (Version 1.2): Population genetics software
for exact tests and ecumenicism. Journal of Heredity 86:248–249.
Reppucci, J., B. Gardner, and M. Lucherini. 2011. Estimating detection and density of the
Andean Cat in the high Andes. Journal of Mammalogy 92:140–147.
Riley, S.P.D., R.M. Sauvajot, T.K. Fuller, E.C. York, D.A. Kamradt, C. Bromley, and R.K.
Wayne. 2003. Effects of urbanization and habitat fragmentation on Bobcats and Coyotes
in southern California. Conservation Biology 17:566–576.
Riley, S.P.D., J.P. Pollinger, R.M. Sauvajot, E.C. York, C. Bromley, T.K. Fuller, and R.K.
Wayne. 2006. A southern California freeway is a physical and social barrier to gene flow
in carnivores. Molecular Ecology 15:1733–1741.
Robinson, K.J.K. 2005. Scat identification and dietary trends of Coyote (Canis latrans),
Gray Fox (Urocyon cinereoargenteus), and Red Fox (Vulpes vulpes) in a mid-Atlantic
ecosystem. M.Sc. Thesis. George Mason University, Fairfax, VA.
Rose, M.D., and G.A. Polis. 1998. The distribution and abundance of Coyotes: The effects
of allochthonous food subsidies from the sea. Ecology 79:998–1007.
Roy, M.S., E. Geffen, D. Smith, E.A. Ostrander, and R.K. Wayne. 1994. Pattern of differentiation
and hybridization in North American wolflike canids, revealed by analysis of
microsatellite loci. Molecular Biology and Evolution 11:553–570.
Northeastern Naturalist Vol. 22, No. 1
C.A. Bozarth, B. Gardner, L.L. Rockwood, and J.E. Maldonado
2015
161
Royle, J.A., and K.G. Young. 2008. A hierarchical model for spatial capture–recapture data.
Ecology 89:2281–2289.
Royle, J.A., R.M. Dorazio, and W.A. Link. 2007. Analysis of multinomial models with
unknown index using data augmentation. Journal of Computational and Graphical Statistics
16:67–85.
Royle, J.A., K.U. Karanth, A.M. Gopalaswamy, and N.S. Kumar. 2009. Bayesian inference
in camera-trapping studies for a class of spatial capture–recapture models. Ecology
90:3233–3244.
Royle, J.A., A.J. Magoun, B. Gardner, P. Valkenburg, and R.E. Lowell. 2011. Density estimation
in a Wolverine population using spatial capture–recapture models. Journal of
Wildlife Management 75:604–611.
Royle, J.A., R.B. Chandler, R. Sollmann, and B. Gardner. 2014. Spatial Capture–Recapture.
Academic Press, Waltham, MA. 577 pp.
Ruell, E.W., S.P.D. Riley, M.R. Douglad, J.P. Pollinger, and K.R. Crooks. 2009. Estimating
Bobcat population sizes and densities in a fragmented urban landscape using noninvasive
capture–recapture sampling. Journal of Mammalogy 90:129–135.
Russell, R.E., J.A. Royle, R. Desimone, M.K. Schwartz, V.L. Edwards, K.P. Pilgrim, K.S.
McKelvey. 2012. Estimating abundance of Mountain Lions from unstructured spatial
samples. Journal of Wildlife Management 76:1551–1561.
Sacks, B.N., B.R. Mitchell, C.L. Williams, and H.B. Ernest. 2005. Coyote movements
and social structure along a cryptic population genetic subdivision. Molecular Ecology
14:1241–1249.
Sacks, B.N., D.L. Bannasch, B.B. Chomel, and H.B. Ernest. 2008. Coyotes demonstrate
how habitat specialization by individuals of a generalist species can diversify populations
in a heterogenous ecoregion. Molecular Biology and Evolution 25:1384–1394.
Samuel, M.D., and E.O. Garton. 1985. Home range: A weighted-normal estimate and tests
of underlying assumptions. Journal of Wildlife Management 49:513–519.
Smallwood, K.S., and C. Schonewald. 1998. Study design and interpretation of mammalian
carnivore-density estimates. Oecologia 113:474–491.
Smith, D.A., K. Ralls, B. Davenport, B. Adams, and J.E. Maldonado. 2001. Canine assistants
for conservationists. Science 291:435.
Smith, D.A., K. Ralls, A. Hurt, B. Adams, M. Parker, B. Davenport, M.C. Smith, and J.E.
Maldonado. 2003. Detection and accuracy rates of dogs trained to find scats of San Joaquin
Kit Foxes (Vulpes macrotis mutica). Animal Conservation 6:339–346.
Smith, D.A., K. Ralls, A. Hurt, B. Adams, M. Parker, and J.E. Maldonado. 2006. Assessing
reliability of microsatellite genotypes from Kit Fox fecal samples using genetic and GIS
analyses. Molecular Ecology 15:387–406.
Smith, W.P. 1983. A bivariate normal test for elliptical home-range models: Biological implications
and recommendations. Journal of Wildlife Management 47:613–619.
Sollmann R., B. Gardner, and J.L. Belant. 2012. How does spatial study design influence
density estimates from spatial capture-recapture models? PLoS O NE 7:e34575.
Spiering, P.A., M.J. Somers, J.E. Maldonado, D.E. Wildt, and M. Szykman Gunther. 2010.
Reproductive sharing and proximate factors mediating cooperative breeding in the
African Wild Dog (Lycaon pictus). Behavioral Ecology and Sociobiology 64:583–592.
Springer, J.T., and C.R. Wenger. 1981. Interactions between and some ecological aspects
of Coyotes and Mule Deer in central Wyoming. Wyoming Game and Fish Publication.
Casper, WY.
Sun, C.C., A.K. Fuller, and J.A. Royle. 2014. Trap configuration and spacing influences
parameter estimates in spatial capture–recapture models. PLoS ONE 9:e88025.
Northeastern Naturalist
162
C.A. Bozarth, B. Gardner, L.L. Rockwood, and J.E. Maldonado
2015 Vol. 22, No. 1
Taberlet, P., S. Griffin, B. Goossens, S. Questiau, V. Manceau, N. Escaravage, L.P. Waits,
and J. Bouvet. 1996. Reliable genotyping of samples with very l ow DNA quantities using
PCR. Nucleic Acids Research 24:3189–3194.
Tigas, L.A., D.H. Van Vuren, and R.M. Sauvajot. 2002. Behavioral responses of Bobcats
and Coyotes to habitat fragmentation and corridors in an urban environment. Biological
Conservation 108:299–306.
Valière, N. 2002. GIMLET: A computer program for analyzing genetic individual-identification
data. Molecular Ecology Notes 2:377–379.
Valière, N., L. Fumagalli, L. Gielly, C. Miquel, B. Lequette, M. Poulle, J. Weber, R. Arlettaz,
and P. Taberlet. 2003. Long-distance Wolf recolonization of France and Switzerland
inferred from non-invasive genetic sampling over a period of 10 years. Animal Conservation
6:83–92.
Vilà, C., I.R. Amorim, J.A. Leonard, D. Posada, J. Castroviejo, F. Petrucci-Fonseca, K.A.
Crandall, H. Ellegren, and R.K. Wayne. 1999. Mitochondrial DNA phylogeography and
population history of the Grey Wolf, Canis lupus. Molecular Ecology 8:2089–2103.
Way, J.G., I.M. Ortega, and P.J. Auger. 2002. Eastern Coyote home range, territoriality, and
sociality on urbanized Cape Cod. Northeast Wildlife 57:1–17.
Way, J.G., L. Rutledge, T. Wheeldon, and B.N. White. 2010. Genetic characterization of
Eastern “Coyotes” in Eastern Massachusetts. Northeastern Naturalist 17:189–204.
Wheeldon, T., B.R. Patterson, and B.N. White. 2010. Colonization history and ancestry of
northeastern Coyotes. Biology Letters 6:246–247.
Windberg, L.A., and F.F. Knowlton. 1988. Management implications of Coyote-spacing
patterns in southern Texas. Journal of Wildlife Management 52:632–640.
Windberg, L.A., S.M. Ebbert, and B.T. Kelly. 1997. Population characteristics of Coyotes
(Canis latrans) in the Northern Chihuahuan Desert of New Mexico. American Midland
Naturalist 138:197–207.