Systematic Variation in Roof Spontaneous Vegetation:
Residential “Low Rise” Versus Commercial “High Rise”
Buildings
Michael L. McKinney and Nicholas D. Sisco
Urban Naturalist, Special Issue No. 1 (2018): 73–88
Full-text pdf.
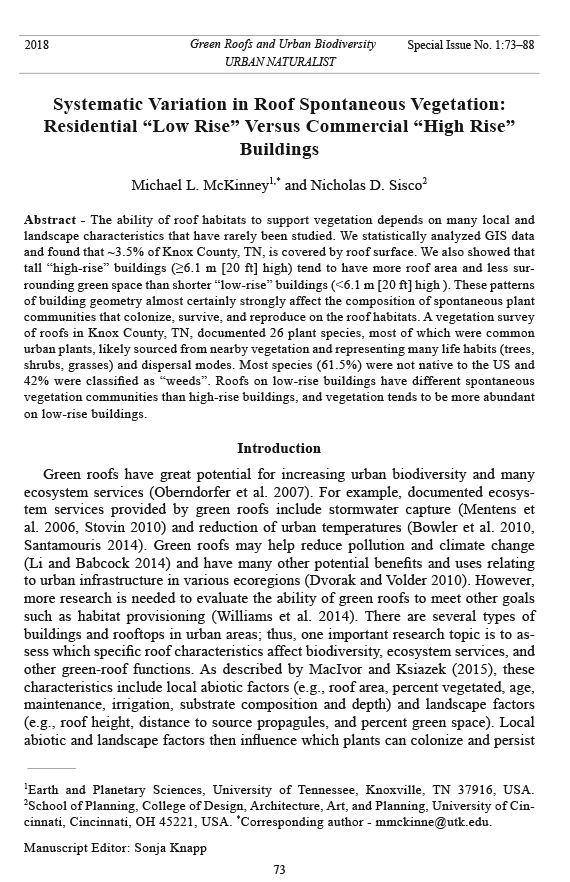
Site by Bennett Web & Design Co.
Urban Naturalist
73
M.L. McKinney and N.D. Sisco
2018 Special Issue No. 1
URBAN NATURALIST
2018 Special Issue No. 1:73–88
Systematic Variation in Roof Spontaneous Vegetation:
Residential “Low Rise” Versus Commercial “High Rise”
Buildings
Michael L. McKinney1,* and Nicholas D. Sisco2
Abstract - The ability of roof habitats to support vegetation depends on many local and
landscape characteristics that have rarely been studied. We statistically analyzed GIS data
and found that ~3.5% of Knox County, TN, is covered by roof surface. We also showed that
tall “high-rise” buildings (≥6.1 m [20 ft] high) tend to have more roof area and less surrounding
green space than shorter “low-rise” buildings (less than 6.1 m [20 ft] high ). These patterns
of building geometry almost certainly strongly affect the composition of spontaneous plant
communities that colonize, survive, and reproduce on the roof habitats. A vegetation survey
of roofs in Knox County, TN, documented 26 plant species, most of which were common
urban plants, likely sourced from nearby vegetation and representing many life habits (trees,
shrubs, grasses) and dispersal modes. Most species (61.5%) were not native to the US and
42% were classified as “weeds”. Roofs on low-rise buildings have different spontaneous
vegetation communities than high-rise buildings, and vegetation tends to be more abundant
on low-rise buildings.
Introduction
Green roofs have great potential for increasing urban biodiversity and many
ecosystem services (Oberndorfer et al. 2007). For example, documented ecosystem
services provided by green roofs include stormwater capture (Mentens et
al. 2006, Stovin 2010) and reduction of urban temperatures (Bowler et al. 2010,
Santamouris 2014). Green roofs may help reduce pollution and climate change
(Li and Babcock 2014) and have many other potential benefits and uses relating
to urban infrastructure in various ecoregions (Dvorak and Volder 2010). However,
more research is needed to evaluate the ability of green roofs to meet other goals
such as habitat provisioning (Williams et al. 2014). There are several types of
buildings and rooftops in urban areas; thus, one important research topic is to assess
which specific roof characteristics affect biodiversity, ecosystem services, and
other green-roof functions. As described by MacIvor and Ksiazek (2015), these
characteristics include local abiotic factors (e.g., roof area, percent vegetated, age,
maintenance, irrigation, substrate composition and depth) and landscape factors
(e.g., roof height, distance to source propagules, and percent green space). Local
abiotic and landscape factors then influence which plants can colonize and persist
1Earth and Planetary Sciences, University of Tennessee, Knoxville, TN 37916, USA.
2School of Planning, College of Design, Architecture, Art, and Planning, University of Cincinnati,
Cincinnati, OH 45221, USA. *Corresponding author - mmckinne@utk.edu.
Manuscript Editor: Sonja Knapp
Green Roofs and Urban Biodiversity
Urban Naturalist
M.L. McKinney and N.D. Sisco
2018 Special Issue No. 1
74
on the roof habitat, and these plants strongly influence the animal community that
subsequently develops (Faeth et al. 2011).
Put another way, green roofs act as filters whereby the habitat properties of
each roof, along with the properties of the surrounding landscape, determine which
groups of organisms can colonize (or be cultivated), persist, and reproduce (Madre
et al. 2014). Spontaneous vegetation—plants that are dispersed to and grow on
green roofs—are excellent indicators of the types of plants that can potentially
colonize and sometimes, persist and reproduce on specific habitats (Archibold and
Wagner 2007, Jim and Chen 2011, Madre et al. 2014). These roof colonizers are
quite diverse (Dunnett 2015) and often provide many ecosystem services and ecological
functions (Del Tredici 2010, Robinson and Lundholm 2012).
Any complete estimate of green-roof habitat in an urban area must therefore
include as many of these local and landscape variables as possible. It is well known
that urban infrastructure is the template that shapes urban ecological processes
at many scales (Forman 2014), and we know that many factors influence that infrastructure.
For example, building age and construction type can affect both the
appropriateness of retrofitting buildings with green roofs and the type of green roof
that can be safely installed (Castleton et al. 2010).
Here, we examine how spatial building geometry affects the biodiversity and
ecology of unmanaged green roofs. Our basic hypothesis is that spatial associations
relating to building geometry are non-randomly distributed in urban space. For example,
taller buildings also tend to have greater roof area because of scaling effects
(e.g., taller buildings need larger foundations, and therefore, greater roof size) and
functional reasons (e.g., taller buildings are often intended to have more volume so
that roof area is increased as well as height). Furthermore, we suggest that spatial
associations among these traits arise because, for example, large commercial buildings
tend to be clustered into localized commercial parts of the urban area, whereas
smaller residential buildings are clustered into localized residential areas.
In addition, we hypothesize that these structural and spatial relationships will
translate into nonrandom patterns on rooftop biological communities, in this case,
spontaneous vegetation. For example, it has been shown that both roof height
(MacIvor 2016) and green-roof substrate depth (Gabrych et al. 2016) influence
insect and plant communities, respectively. As potential habitats, larger buildings
tend to have more roof area with more structural support for deeper, moister, growing
substrates with more moderate temperatures than smaller buildings, which have
less roof area and thinner growing-substrates. In terms of dispersal potential, larger
buildings will have different landscape characteristics than smaller ones, and higher
roofs may be surrounded by less green space, which makes plant and animal dispersal
to roofs on larger buildings more difficult and thus less likely.
Methods
We measured several aspects of building geometry (such as building height,
roof area, and surrounding green space) and related these to spontaneously growing
plant communities that were found on the roofs of surveyed buildings. Our study
Urban Naturalist
75
M.L. McKinney and N.D. Sisco
2018 Special Issue No. 1
focused on Knox County, TN, USA, which contains the rapidly growing city of
Knoxville. Settled in 1792, Knoxville has a population of 184,281 and is the 3rdlargest
city in the state (US Census Bureau 2015). Due to its moderate climate and
proximity to a major national park, the rapid growth of Knoxville is typical of many
cities in the Southeastern US that are characterized by extensive urban sprawl (Cho
et al. 2010).
Knox County has no cultivated or maintained green roofs, so our survey focused
on spontaneous vegetation that was found growing on roofs (usually abandoned
structures) that were not designed specifically as vegetative habitat. This site
characteristic is a critical point, expanded on below, because our findings focus
on which plants can disperse to and germinate on these roof habitats; they cannot
address how many of these plants can survive and reproduce over a long period,
compared to constructed green roofs that are specifically designed and maintained
as plant habitats (e.g., as described by Oberndorfer et al. 200 7).
Geospatial data
We acquired the geospatial data used to conduct this research from the Knoxville
Geographic Information Systems (KGIS) group from the year 2010. The shapefiles
included: building footprints, roads (edge of pavement), structures, water, and
county/city boundaries. In 2010, there were ~230,000 buildings in Knox County.
We conducted all geospatial analyses in ESRI ArcGIS software. We projected the
data in Tennessee State Plane (feet) and then converted to metric values. The building-
footprint shape-file displays every building in Knox County as rooftop shapes
traced from aerial imagery.
In order to process such a large dataset, we used the grid-index features tool to
divide Knox County into 1-km2 cells, which produced a total of 1232 cells (Fig. 1).
Building footprints were added to the map and were spliced by the grid lines to accurately
measure roof area and percent roof-cover per cell. After using the intersect
tool to splice the polygons, we recalculated the geometry to obtain area (m2) per
roof. We used the dissolve tool to create a single multipart shapefile per cell, which
aggregated the total roof-area per cell. We divided roof area by the area of the cell
(1 km2) to calculate the percent roof-cover per cell and for all of Knox County. The
individual area of each roof was also calculated and exported i nto an excel file.
In addition to roof area, we used the KGIS database to analyze roof height.
Height for each building is an attribute calculated by KGIS prior to our analysis.
This database also provided information on nearest-neighbor distance, which was
calculated as the shortest distance between each building and the closest adjacent
building. Finally, we calculated green space per cell by creating a shape file of impervious
and non-vegetated objects by merging the transportation shape-file (which
included roads, bridges, and driveways) with the building footprints shape-file, the
miscellaneous structures shape-file (for example, storage tanks and other non-vegetated
objects), and the hydrological shape file (creeks, rivers, lakes). We dissolved
and clipped per sample cell the non-vegetated shape file in order to complete the
geoprocessing in an efficient and timely manner. We then figured green-space area
using basic geometry calculations.
Urban Naturalist
M.L. McKinney and N.D. Sisco
2018 Special Issue No. 1
76
We employed distributional statistics (central tendencies, skewness, histograms)
and bivariate and multivariate methods to analyze the data on roof area, roof height,
nearest neighbor, and green space for each cell to identify any relationships among
them. All analyses were run in SAS software, version 9.0 (SAS 2016). For multivariate
analysis, we utilized principle component and factor analysis to examine
any covariations between building height, area, nearest neighbor, and surrounding
green space. Our goal was to quantify any non-random patterns in building geometry.
Where needed, we normalized the per-cell data using log transformation.
We log10-transformed all variables except percent green space for the correlation
analysis of the 1232 cells to meet the conditions of normality.
We compared patterns of roof area, height, nearest neighbor, and adjacent
green space in high-rise buildings versus low-rise buildings for the multivariate
cell-analysis and the vegetation survey. We chose the 6.1-m (20-ft) cut-off to differentiate
low- and high-rise buildings because residential homes are typically 2
stories or less, which usually translates into a height of ≤6.1 m (20 ft) (http://www.
knoxcounty.org/codes/). In contrast, buildings taller than 6.1 m (20 ft) tend to be
larger commercial buildings (e.g., apartments, offices, retail) not found in typical
mass suburban-housing subdivisions.
We derived a subset from the KGIS data (not based on per-cell data) of roof area,
height, and green space to perform a finer-scale analysis. We employed the subset
features tool (ArcGIS) to select 100 randomly sampled buildings and create a 3.05-m
(10-ft) buffer around each building. The impervious surface shapefile was then
Figure 1. Example of a 1-km2 cell showing University of Tennessee campus (including
football stadium.) Dark areas are building footprints (roof areas). Gray shading indicates
impervious and other non-vegetated areas. White areas represent vegetation (green space).
This cell (Cell 902) has 19.0% roof cover.
Urban Naturalist
77
M.L. McKinney and N.D. Sisco
2018 Special Issue No. 1
intersected and clipped to the 3.05-m (10-ft) buffer. We used the buffer to measure the
percentage of green space around each of the chosen buildings (Fig. 2). These data
were analyzed using the bivariate and multivariate methods described above to examine
finer-scale relationships among roof area, height, and green space.
Spontaneous vegetation survey
To directly examine the effects of roof area, height, and surrounding green space
on roof vegetation, we surveyed spontaneous vegetation growing on roof habitats.
Based on our analysis of the KGIS data, we selected 12 high-rise and 12 low-rise
cells for subsequent survey. We surveyed all 24 cells between 3 April 2015 and 12
July 2015 to locate as many plants as possible growing on building roofs in those
cells. Our surveys included habitats on any roof structures that occurred at or above
roof level, such as rain gutters and chimneys. There are no designed or cultivated
green roofs in Knox County; thus, these plants were generally growing in locations
where soil had somehow accumulated naturally, a process which occurs through
time (Getter et al. 2007). We used a laser distance-meter with a 200-m (656-ft)
range (Leica Disto E7500i meter, Leica Geosystems, Norcross, GA) to measure the
building height of buildings with vegetation.
We followed 2 basic survey methods. For buildings with roof tops visible from
the ground, such as most residential houses, we could often confirm plant presence
with walking or driving surveys. However, for high-rise buildings, we had to rely
Figure 2. 3.05-m(10-ft) buffer area denoted by gray line. We subtracted the area of impervious
surface (striped) from the total buffer area to derive green-space area within the buffer
zone and converted this value to a percentage. In this case: buffer area (382.80 m2) - impervious
area (248.71m2) = green-space area (134.09 m2). Green-space percentage = green space
area/buffer area = 35.03%.
Urban Naturalist
M.L. McKinney and N.D. Sisco
2018 Special Issue No. 1
78
on observations from elevated areas, which often included gaining access to rooftops
of commercial buildings to examine them and to view surrounding roof tops.
When we observed plants growing on rooftops, we typically gained access to the
roof to identify or collect them directly. In some cases, however, especially for very
tall buildings or derelict buildings with unsafe access, we used telephoto lenses to
obtain photographs that we used for identification. In an effort to standardize for
sampling effort, we devoted roughly 18 person-hours to each of the 24 cells.
Our roof survey encountered 2 basic types of roof habitats that supported spontaneous
plants. All of the houses surveyed had angled roofs constructed of asphalt
roof shingles. In these cases, plants were growing in crevices between the shingles,
most often around or on a chimney, and (commonly) in gutters. We sometimes
observed vines growing on the shingle exterior. The second type of roof habitat
commonly found was a flat-top roof typical on commercial buildings. Surfaces
of these roofs were paved with concrete, asphalt, or pebble-covered tar paper. We
documented plants on these flat roofs in many areas, usually where some sediment
accumulation had occurred in the corners or depressions on the roof. Both of these
roof habitats have characteristics that are very different from those of designed
green-roof habitats, which has important implications as discussed below.
We consulted the website of the Knox County tax assessor (http://tn-knoxassessor.
publicaccessnow.com/PropertyLookup.aspx) to determine the age of 64
surveyed buildings (year constructed) with spontaneous vegetation. We assessed
the effects of building age on spontaneous vegetation. To specifically detect
if high-rise roofs are proportionately younger or older than low-rise roofs, we
performed a chi-square test using our high- and low-rise definitions; we further
defined a building as old if building age was ≥40 y and as young if building age
was less than 40 y.
We compared plant species occurrence on high-rise buildings versus low-rise
buildings. To reduce the statistical “noise”, we excluded from analysis species with
fewer than 2 total occurrences. We used the weediness, life-habit, and nativity designations
of all species found as listed by the USDA (www.usda.gov). We obtained
seed-dispersal information from the Dispersal and Diaspore Database (Hintze et
al. 2013). Weeds are defined here as those plants causing significant economic or
ecological impacts. We define native species as those occurring in North America
before European colonization. Using bivariate and multivariate factor analysis,
we examined the effects of building height, building area, and green space on the
composition of plant associations found on the buildings we surveyed.
Results
Roof cover in each 1-km2 cell studied in Knox County, TN, ranged from much
less than 1% to over 22% (Fig. 3). The sum of the roof cover for all cells is 47.72
km2, indicating that ~3.5% of Knox County was covered by roof area (47.72 km2
roof cover/1362 km2 total area). The densest roof cover is in historic downtown
Knoxville in the county center and trends SW along the interstate-highway corridor
(Fig. 3). Cells in the urban core had the highest roof cover, with a maximum
Urban Naturalist
79
M.L. McKinney and N.D. Sisco
2018 Special Issue No. 1
of 22.7% and an average for the urban core of roughly 16% roof cover. Cells in the
suburban areas typically averaged around 11% roof cover but varied from 4% to
17%, depending on the specific housing subdivisions analyzed. Cells sampled in
rural parts of the county, far from the urban core, varied from 0% to 7% roof cover,
with an average of ~2%.
The frequency distribution of roof area in highly urban (downtown) cells shows
a strongly right-skewed distribution (median = 177.93 [SD = 98.44], skewness =
4.62 [SE = 0.120], kurtosis = 33.04 [SE = 0.240]). This result indicates that, for
highly urban areas, available roof area consists of many small roofs and a few very
large roof tops. In suburban cells, the frequency distribution of roof area is less
right-skewed and reflects decreased presence of large buildings (median = 253.87
[SD = 88.63], skewness = 2.50 [SE = 0.364], kurtosis = 14.98 [SE = 0.364]).
Correlation analysis showed that percent green space was significantly negatively
correlated with both median roof height and median roof area for the cells
Figure 3. Knox County, TN: Percent roof cover in each 1-km2 cell ranges from much less than
1% to over 22%. Cumulative roof cover for the County is about 3.5% of total county area.
Table 1. Correlation matrix of median variables for 1232 cells. All variables except percent green space
are log10 transformed. Roof height = distance from the ground to the roof, roof area = area of roof top,
percent greenspace = percentage area per cell covered with vegetation, and nearest neighbor = distance
to nearest building. * indicates values that are different from 0 at a significance level alpha = 0.05
Variables Roof height Roof area Percent Greenspace Nearest neighbor
Roof height 1*
Roof area 0.756* 1*
Percent greenspace -0.411* -0.472* 1*
Nearest neighbor -0.012 0.048 0.131 1*
Urban Naturalist
M.L. McKinney and N.D. Sisco
2018 Special Issue No. 1
80
(Table 1). Also, median roof area and median roof height were strongly positively
correlated (Table 1). Nearest-neighbor analysis showed no significant relationships
(Table 1) among any of the variables studied here.
Analysis of the 100 randomly selected buildings also indicated a strong positive
relationship between roof area and roof height (Fig. 4A). There is some evidence
that the relationship is curvilinear, as demonstrated by the better fit of the power
function. Also, both roof area and roof height showed a strong negative and curvilinear
relationship to percent green buffer (Fig. 4).
Figure 4. (A) Positive relationship between roof height and roof area for 100 randomly selected
buildings (y = 0.936x1.66, R² = 0.636, P < 0.01). Note negative relationship between
percent green-buffer percentage (x 10 for visual display on graph) and roof height for 100
randomly selected buildings (y = 0.495x2 - 30.338x + 895.42, R² = 0.279, P < 0.01). (B)
Negative relationship between percent green-buffer and roof area for 100 randomly selected
buildings (y = 155.13x-0.234, R² = 0.422, P < 0.01).
Urban Naturalist
81
M.L. McKinney and N.D. Sisco
2018 Special Issue No. 1
For the 1232 cells, a principal component analysis (PCA) summarized these
patterns with roof area and roof height loading very highly (0.614, 0.597, respectively)
on Axis 1, with green space (- 0.515) showing an opposite pattern (Fig. 5).
Overall, Axis 1 explains over 50% (51.8%) of the variation in the data. Roof nearest
neighbor shows no consistent relationship to those variables and loads very highly
on Axis 2 (0.973), explaining 26.0% of the total variation. A plot of factor scores
of all 1232 cells onto Axis 1 and Axis 2 shows that high-rise cells form a separate
population from low-rise cells (Fig. 5). The latter are characterized by lower roofarea
and height and greater green space (because they score lower on Axis 1, which
is negatively correlated with green space).
The spontaneous vegetation survey found 26 plant species with an occurrence
>1. These 26 species represent a wide variety of life habits; 38.5% were native,
61.5% were non-native, 42.3% were weeds, and 57.7% were non-weeds. Of these,
34.6% were animal dispersed and 65.4% were mechanically dispersed (Table 2). A
plot of the factor loadings for the plants co-occurring in the 24 cells indicates which
plants tend to occur together: species to the right are increasingly associated with
Figure 5. Plot of factor scores of all 1232 cells onto Axis 1 and Axis 2. For this PCA, roofarea
and roof-height loading vary highly (0.614, 0.597, respectively) on Axis 1, with green
space (- 0.515) showing an opposite pattern. Centroid envelope encompasses 95% of score
values for respective category. The major axis (1) contains over half of the variation and
shows the high covariation of median roof area with median roof height and the negative
relationship of those 2 variables with greenspace.
Urban Naturalist
M.L. McKinney and N.D. Sisco
2018 Special Issue No. 1
82
Table 2. Plants identified in this study, with various attributes shown. Weed, growth habit, and nativity status from USDA classifications (www.usda.gov).
Seed-dispersal information was obtained from the Dispersal and Diaspore Database (Hintze et al. 2013). Native = native to US. High- or low-rise trend
derived from Figure 6 as explained in text. * = listed as common spontaneously growing urban plants of the northeastern US (Del Tredici 2010).
Species Common name Abundance Life habit Nativity Weed Dispersal
High-rise trend
Ailanthus altissima (Mill.) Swingle* Tree of Heaven 22 Tree Non Y Mechanical
Ambrosia artemisiifolia* Besser Ragweed 17 Herb Native Y Mechanical
Buddleja davidii Franch. Butterfly Bush 6 Shrub Non Y Mechanical
Ligustrum sinense Lour.* Chinese Privet 19 Shrub Non Y Animal
Paulownia tomentosa (Thunb.) Steud.* Princess Tree 19 Tree Non Y Mechanical
Pellaea atropurpurea (L.) Link Cliff Fern 4 Fern Native N Mechanical
Phytolacca americana* L. Pokeweed 14 Herb Native N Animal
Solidago canadensis* L. Canada Goldenrod 14 Herb Native N Mechanical
Low-rise trend
Acer saccharinum* L. Silver Maple 4 Tree Native N Mechanical
Cercis canadenisis L. Redbud 7 Tree Native N Mechanical
Daucus carota* L. Wild Carrot 10 Herb Non Y Mechanical
Parthenocissus quinquefolia* (L.) Planch. Virginia Creeper 7 Vine Native N Animal
Plantago major* L. Common Plantain 19 Grass Non N Animal
Pyrus calleryana Decne. Bradford Pear 8 Tree Non N Animal
Rubus allegheniensis* Porter Blackberry 11 Shrub Native N Animal
Setaria faberi* R.A.W. Herrm. Giant Foxtail 11 Grass Non Y Mechanical
Taraxacum officinale* F.H. Wigg. Dandelion 22 Herb Non N Mechanical
No clear trend
Asplenium platyneuron D.C. Eaton Ebony Spleenwort 4 Fern Native N Mechanical
Campsis radicans (L.) Bureau Trumpet Vine 18 Vine Native N Mechanical
Cirsium vulgare* (Savi) Ten. Bull Thistle 4 Herb Non Y Mechanical
Hedera helix Lowe English Ivy 18 Vine Non Y Animal
Lonicera japonica* Thunb. Japanese Honeysuckle 13 Vine Non Y Animal
Morus alba* Sudw. White Mulberry 13 Tree Non N Animal
Paspalum dilatatum Poir. Dallisgrass 13 Grass Non N Mechanical
Urtica dioica* L. Stinging Nettle 2 Herb Non N Mechanical
Verbascum thapsus* L. Great Mullein 3 Herb Non Y Mechanical
Urban Naturalist
83
M.L. McKinney and N.D. Sisco
2018 Special Issue No. 1
high-rise buildings and species to the left are increasingly associated with low-rise
buildings (Fig. 6). A plot of the factor scores of those plants indicates that plant species
assemblages on high-rise buildings seem to differ (have little overlap) relative
to the assemblages on low-rise buildings (Fig. 6).
Table 2 shows the 26 species on the basis of whether they occur mostly on lowrise
(loadings <- 0.3 on Fig. 6) or high rise buildings (loadings >0.3 on Fig. 6). Ten
species (38.5%) are native to the US, 11 species (42.3%) are classified as weeds,
and 17 species (65.4%) are considered common urban plants in North America (Del
Tredici 2010). Our analyses identified no association between height and life history,
nativity, weed status, or main dispersal mode. The sample size was relatively
small for each category (9–11, out of 26 total); thus, we performed a Fisher exact
probability test to relate each of the 4 attributes to high- or low-rise status. None of
the attributes were non-randomly distributed at the 0.05 level of confidence.
Analyses of the vegetation-survey data identified a strong effect of building
height on plant abundance and plant species richness (Fig. 7). Plant abundance is
the total number of occurrences (observations) for all plants on all roofs at each
height interval. For example, interval 10 = total occurrences at 0–3.05 m (0–10 ft),
interval 20 = total occurrences at 3.06–6.1 m (>10 to 20 ft.) and so on. Similarly,
Richness equals the total number of species found for all plants on all roofs at each
interval. There is a roughly exponential decline in both abundance and richness
with increasing building height (Fig. 7).
Figure 6. Plot of factor loadings for plant species on F1 and F2, based on their co-occurrences
on the 24 cells that were surveyed. Species to the right are increasingly associated with
high-rise buildings. Species to the left are increasingly associated with low-rise buildings.
Urban Naturalist
M.L. McKinney and N.D. Sisco
2018 Special Issue No. 1
84
Regarding the effects of building age on spontaneous vegetation, regression
of building age (y) onto building height indicated no relationship (n = 64, R2 =
0.004, F = 0.001, P = 0.976). Multiple regression of plant species richness onto
age (y) and building height (m) indicated that both had an effect on species richness:
Richness = - 0.0146Height + 0.061Age + 2.75 (n = 64, adj R2 = 0.683, F =
68.81, P < 0.0001). Richness increased with age but declined with height. Multiple
regression of plant species abundance onto age (y) and building height (m)
indicated that both had an effect on abundance: Abundance = - 0.0232Height +
0.073Age + 4.95 (n = 64, adj R2 = 0.702, F = 75.07, P < 0.0001). Abundance increased
with age but declined with height.
The chi-square distribution for the 64 buildings of known age was as follows:
old/low rise = 11, old/high-rise = 12, young/low-rise = 18, young/high-rise = 23.
The results are: chi-square = 0.092 (critical value = 3.841), P = 0.762 with alpha
= 0.05. The computed P-value is greater than the significance level alpha = 0.05;
thus, one cannot reject the null hypothesis H0 that the rows and columns are independent,
i.e., there is no apparent pattern in age distribution between high-rise
and low-rise buildings.
Discussion
The KGIS data indicated that, for all of the total area of Knox County, there
is a great deal of potential green-roof habitat, with a total roof area that exceeds
3.5% of the Knox County (Fig. 3). This finding is significant because Knoxville is
Figure 7 Plant species abundance and species richness decline w ith building height.
Urban Naturalist
85
M.L. McKinney and N.D. Sisco
2018 Special Issue No. 1
typical of many cities in the US, especially in the southeastern US, in experiencing
substantial urban sprawl (Cho et al. 2010). This form of growth is notorious for its
negative environmental impacts via extensive devegetation and habitat loss (Martinuzzi
et al. 2015). That such a large area (3.5%) of the Knox County is covered by
roof area implies there is a very significant amount of potentially available habitat
that could mitigate the effects of sprawl on biological systems.
In addition, we showed that this potential green-roof habitat differs systematically
on the basis of height, area, and surrounding green space. Specifically, tall
high-rise buildings tend to have more roof area and less green space compared
to shorter low-rise buildings (Figs. 4 and 5). Also, the distribution of green-roof
habitat is variable; highly urbanized downtown areas have many smaller buildings
interspersed with relatively few very large roofs. Roof area in more suburban areas
tends to be somewhat more evenly distributed (less right skewed ).
These building and roof patterns have significant implications for the plant communities
that can colonize, survive, and reproduce on these habitats. Our tentative
findings from the plant surveys on roof tops indicate that different communities are
found on the taller high-rise buildings than on the low-rise roof tops (Fig. 6). We
did not, however, find any clear trend in life history, nativity, dispersal mode, or
weediness among these 2 communities (Table 2).
Our findings also indicate that taller buildings have lower plant abundance and
lower plant species richness (Fig. 7). Increasing roof height will, therefore, shape
green-roof plant communities by reducing the number of species that can disperse to
high-rise sites. Madre et al. (2014), for example, found that roof height was a significant
factor in shaping the taxonomic and functional compositions of the colonizing
plant communities of 115 green roofs in northern France. Our finding that increasing
roof height reduced spontaneous plant diversity and abundance was also shown for
bees and wasps (MacIvor 2016) and for arboreal flora in Hong Kong (Jim and Chen
2011). As previously documented by Liu et al. (2016) for other urban areas, we found
that percent green space is negatively related to building height. For bees and wasps,
reduced surrounding ground-level green space is related to decreasing species richness
and abundance on vegetated roofs (MacIvor and Ksiazek 2015, Tonietto et al.
2011). Our results also indicate that older buildings tend to have more roof vegetation,
a pattern also noted by Jim and Chen (2011) in Hong Kong.
Importantly, our study does not directly address the ability of the spontaneous
vegetation found here to survive and reproduce on roofs over the long term. Many
of the plants observed (especially trees and shrubs) were in the early stages of
growth and we do not know if they could survive for many years and reproduce on
roof habitats where soil was sometimes much thinner than on the ground. Indeed,
Miller et al. (2014) found that most rooftop tree seedlings did not survive for more
than 1 year. Furthermore, the survival and reproductive potential of these species
will likely be different for plants on designed green-roof habitats. As described by
Oberndorfer et al. (2007), there are both extensive and intensive designs for such
green roofs. The extensive roof, with an average substrate of 2–20 cm and requiring
little irrigation or maintenance (Oberndorfer et al. 2007), is most similar to the
Urban Naturalist
M.L. McKinney and N.D. Sisco
2018 Special Issue No. 1
86
kinds of neglected roof habitats analyzed here. However, there are still many differences
between designed extensive roofs and the residential and commercial roof
tops studied here. Most notable perhaps is that (where they are not regularly “weeded”)
extensive roofs would likely generally provide better habitat for spontaneous
vegetation because of the relatively less hostile conditions (e.g., better growing
media) of such roofs. Most of the plants in this study were growing in small areas
with sparse soil accumulation and no intentional design for such habitat conditions
as shade, soil thickness, or soil quality (including nutrients and moisture). Several
studies have shown that substrate depth plays a major role in shaping roof-plant
community composition (Brown and Lundholm 2015, Gabrych et al. 2016, Ksiazek
et al. 2017), including colonizing plant communities (Madre at al. 2014).
Although our findings cannot provide information on which plants can survive
and reproduce on designed green roofs, we suggest that they provide information
on a “first filter” for green-roof communities, that of dispersal and germination.
That is, they shed light on which local plant species may be most capable of dispersing
to these rooftop habitats. For plants that are able to colonize high-rise
habitats via natural dispersal or cultivation, our results show they will likely find
more roof area for growth and reproduction than in residential and other low-rise
roof habitats. Roof area has been shown to affect roof-community composition
in spontaneous vegetation (Madre et al. 2014). In addition to more area, roofs on
larger buildings have greater structural support with the potential to hold much
deeper soils.
We cannot yet verify specific attributes (life habit, nativity, weed status, and dispersal
mode) that can explain why certain plant species tend to colonize and grow
on the roofs studied here. However, there are some general patterns about these roof
colonizers that we hope will stimulate further research. One pattern is that roughly
two-thirds (65.4%) of the spontaneous vegetation was composed of plants that are
common in many urban habitats. These plants are familiar species in many hardscape
and disturbed urban areas, such as roadsides, sidewalk crevices, and vacant
lots, and they are well adapted to the urban landscape with their high tolerance
for dry, hot, and sunny habitats (Archibold and Wagner 2007, Del Tredici 2010).
As argued by Madre et al. (2014), such urban species have value in their ease of
maintenance combined with their performance of ecosystem services and ecological
functions (see also Robinson and Lundholm 2012). Interestingly, although 86%
of their urban roof species were native, we found a much lower percentage (38.5%)
of spontaneous roof species that were native. Another pattern in our study was
the presence of several species that are commonly cultivated in landscaping (e.g.,
Buddleja davidii [Butterfly Bush], Campsis radicans [Common Trumpet Creeper],
Pyrus calleryana [Bradford Pear]) pointing to this activity as a potentially important
avenue for green-roof dispersal. In a study on a Canadian University campus,
Archibold and Wagner (2007) also noted the importance of ornamental species
dispersing to rooftops.
In conclusion, our results support the predictions that building configurations
affecting roof habitats (e.g., roof area, height, and surrounding green space) occur
Urban Naturalist
87
M.L. McKinney and N.D. Sisco
2018 Special Issue No. 1
in non-random spatial associations that produce different communities of spontaneous
vegetation. Our findings also confirm a larger view that recommends that
vegetation on roofs should not be considered in isolation, but should be considered
as part of a much larger “green and gray infrastructure” with a large-scale dynamic
that has many implications for designing sustainable cities (Forman 2014). Clearly,
however, many roof-habitat variables, in addition to those studied here, affect patterns
of dispersal and persistence in roof vegetation. Specific recommendations for
further work include determining survival and reproduction in rooftop spontaneous
vegetation, and making detailed estimates of the ecosystem services provided by
these species.
Acknowledgments
Assistance with plant identification was provided by the University of Tennessee
(UT) Botany Department, especially Aaron Floden, the UT Forestry Department, and the
UT Arboretum.
Literature Cited
Archibold, O.W., and L. Wagner. 2007. Volunteer vascular plant establishment on roofs at
the University of Saskatchewan. Landscape and Urban Planning 79 :20–28.
Bowler, D.E., L. Buyung-Ali, and T.M. Knight. 2010. Urban greening to cool towns and
cities: A systematic review of the empirical evidence. Landscape and Urban Planning
97:147–155.
Brown, C., and J.T. Lundholm. 2015. Microclimate and substrate depth influence greenroof
plant community dynamics. Landscape and Urban Planning 143 :134–142.
Castleton, H.F., V. Stovin, and S.B. Beck. 2010. Green roofs: Building ener gy savings and
the potential for retrofit. Energy and Buildings 42:1582–1591.
Cho, S.H., D.M. Lambert, R.K. Roberts, and S.G. Kim. 2010. Demand for open space and
urban sprawl: The case of Knox County, Tennessee. Pp. 171–193, In A. Paez, J. LeGallo,
R.N. Buliung, and S. Dallerba (Eds.). Progress in Spatial Analysis: Methods and Applications.
Springer-Verlag, Berlin, Germany. 492 pp.
Del Tredici, P. 2010. Wild Urban Plants of the Northeast. Cornell University Press, Ithaca,
NY, USA. 392 pp.
Dunnett, N. 2015. Ruderal green roofs. Ecological Studies 223:2 33–255.
Dvorak, B., and A. Volder. 2010. Green-roof vegetation for North American ecoregions: A
literature review. Landscape and Urban Planning 96:197–213.
Faeth, S.H., C. Bang, and S. Saari. 2011. Urban biodiversity: Patterns and mechanisms.
Annals of the New York Academy of Science 1223:69–81.
Forman, R.T.T. 2014. Urban Ecology. Cambridge University Press, Cambridge, UK.
476 pp.
Gabrych, M., D.J. Kotze, and S. Lehvävirta. 2016. Substrate depth and roof age strongly
affect plant abundances on sedum–moss and meadow green-roofs in Helsinki, Finland.
Ecological Engineering 86:95–104.
Getter, K. L., D.B. Rowe, and J.A. Andresen. 2007. Quantifying the effect of slope on extensive
green-roof stormwater retention. Ecological Engineering 31:225–231.
Hintze, C., F. Heydel, C., Hoppe, S. Cunze, A. König, and O. Tackenberg. 2013. D³: The
dispersal and diaspore database—baseline data and statistics on seed dispersal. Perspectives
on Plant Ecology, Evolution, and Systematics 15:180–192. Available online at
www.seed-dispersal.info. Accessed on 11 November 2016.
Urban Naturalist
M.L. McKinney and N.D. Sisco
2018 Special Issue No. 1
88
Jim, C.Y., and W.Y. Chen. 2011. Bioreceptivity of buildings for spontaneous arboreal flora
in compact city environment. Urban Forestry and Urban Greening 10:19–28.
Ksiazek, K., J. Herrmann, Sean B. Menke, and Manfred Köhler. 2017. If you build it, will
they come? plant and arthropod diversity on urban green roofs over time. Urban Naturalist
Special Issue 1:52–72.
Li, Y.L., and R. W. Babcock. 2014. Green roofs against pollution and climate change: A
review. Agronomy and Sustainable Development 34:695–705.
Liu, Y.Q., Q.Y. Meng, J.H. Zhang, L.L. Zhang, T. Jancso, and R. Vatseva. 2016. An effective
building-neighborhood green-index model for measuring urban green space. International
Journal of Digital Earth 9:387–409.
MacIvor, J.S. 2016. Building height matters: Nesting activity of bees and wasps on vegetated
roofs. Israel Journal of Ecology and Evolution 62:88–96.
MacIvor, J.S., and K. Ksiazek. 2015. Invertebrates on green roofs. Pp. 333–355, In R. Sutton
(Ed.) Green Roof Ecosystems. Springer International Publishing, Cham, Switzerland.
DOI:10.1007/978-3-319-14983-7_14.
Madre, F., A. Vergnes, N. Machon, and P. Clergeau. 2014. Green roofs as habitats for wild
plant species in urban landscapes: First insights from a large-scale sampling. Landscape
and Urban Planning 122:100–107.
Martinuzzi, S.J., J.C. Withey, A.M. Pidgeon, A.J. McKerrow, and V.C. Radeloff. 2015. Future
land-use scenarios and the loss of wildlife habitats in the southeastern United States.
Ecological Applications 25:160–171.
Mentens, J., D. Raes, and M. Hermy. 2006. Green roofs as a tool for solving the rainwater
runoff problem in the urbanized 21st century? Landscape and Urban Planning 77:217–226.
Miller, L.E., A.E. Heim, and J. Lundolm. 2014. Green-roof vegetation type affects germination
and initial survival of colonizing woody species. Urban Forestry and Urban
Greening 13:892–899.
Oberndorfer, E., J. Lundholm, B. Bass, R.R. Coffman, H. Doshi, N. Dunnett, S. Gaffin, M.
Köhler, K.K.Y. Liu, and B. Rowe. 2007. Green roofs as urban ecosystems: Ecological
structures, functions, and services. Bioscience 57:823–833.
Robinson, S.L., and J.T. Lundholm. 2012. Ecosystem services provided by urban spontaneous
vegetation. Urban Ecosystems 15:545–557.
SAS. 2016. Statistical Analysis System, 9.0. Cary, NC.
Santamouris, M. 2014. Cooling the cities: A review of reflective and green-roof mitigation
technologies to fight heat island and improve comfort in urban environments. Solar
Energy 103:682–703.
Stovin, V. 2010. The potential of green roofs to manage urban stormwater. Water and Environment
Journal 24:192–199.
Tonietto, R., J. Fant, J. Ascher, K. Ellis, and D. Larkin. 2011. A comparison of bee communities
of Chicago green roofs, parks, and prairies. Landscape and Urban Planning
103:102–108.
US Census Bureau. 2015. Urban population by state. Available online at http://www.census.
gov/. Accessed 12 March 2016.
Williams, N.S.G., J.T. Lundholm, and J.S. MacIvor. 2014. Do green roofs provide habitat
for biodiversity? Journal of Applied Ecology 51:1643–1649.